Deep hole machining depth optimization method based on iterative learning
A technology of machining depth and iterative learning, applied in the field of deep hole machining depth optimization based on iterative learning, can solve problems such as insufficient prediction accuracy and poor practicability, and achieve the effects of improving prediction accuracy, good practicability, and solving insufficient prediction accuracy.
- Summary
- Abstract
- Description
- Claims
- Application Information
AI Technical Summary
Problems solved by technology
Method used
Image
Examples
Embodiment Construction
[0020] refer to Figure 1-2 . The specific steps of the deep hole processing depth optimization method based on iterative learning in the present invention are as follows:
[0021] 1. Establish a cutting force prediction model for deep hole machining.
[0022] The classic cutting force empirical formula is expressed as the following form
[0023] F(z)=C 0 f a ·s b ·z n
[0024] Among them, the cutting parameters in the model include the feed rate f, the spindle speed s and the cutting depth z; the constant C 0 and the coefficients a, b, n of each cutting parameter are obtained through single factor test calibration respectively. In the case of given feed rate and spindle speed, the empirical model of cutting force in deep hole machining with machining depth as independent variable is expressed as
[0025] F(z)=C·z n
[0026] Among them, the model coefficients C and n are obtained by fitting the experimental data.
[0027] 2. Set the initial processing depth and per...
PUM
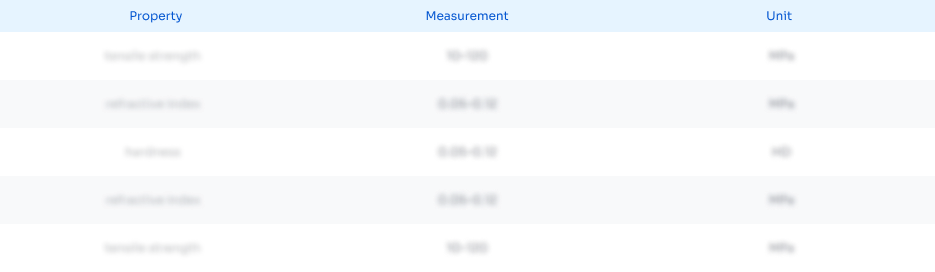
Abstract
Description
Claims
Application Information
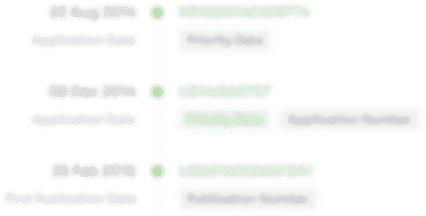
- R&D Engineer
- R&D Manager
- IP Professional
- Industry Leading Data Capabilities
- Powerful AI technology
- Patent DNA Extraction
Browse by: Latest US Patents, China's latest patents, Technical Efficacy Thesaurus, Application Domain, Technology Topic, Popular Technical Reports.
© 2024 PatSnap. All rights reserved.Legal|Privacy policy|Modern Slavery Act Transparency Statement|Sitemap|About US| Contact US: help@patsnap.com