Hyperspectral image robustness classification method based on segmentation depth characteristics and low-rank representation
A hyperspectral image and depth feature technology, applied in the fields of instruments, character and pattern recognition, computer parts, etc., can solve the problems of insufficiently exploiting the spatial correlation of hyperspectral images, limiting the classification accuracy of hyperspectral images, and achieve good classification. effect of effect
- Summary
- Abstract
- Description
- Claims
- Application Information
AI Technical Summary
Problems solved by technology
Method used
Image
Examples
Embodiment Construction
[0030] The present invention proposes a hyperspectral image robust classification method based on segmented depth features and low-rank representation, the specific process is as follows:
[0031]1. Calculate the correlation coefficient between spectra
[0032] Hyperspectral images include hundreds of continuous spectral bands, and because of this continuity, there is a strong correlation between the bands. In order to better explore the correlation of different spectral regions, the correlation coefficient between different band spectra of hyperspectral images is calculated, and then the connected spectral bands with correlation coefficients greater than 0 are divided into one section, and then the original hyperspectral image is divided into spectral dimensions segment.
[0033] 2. Training stack denoising autoencoder
[0034] First, the hyperspectral image pixels are randomly divided into training data and test data, and according to the spectral dimension division method...
PUM
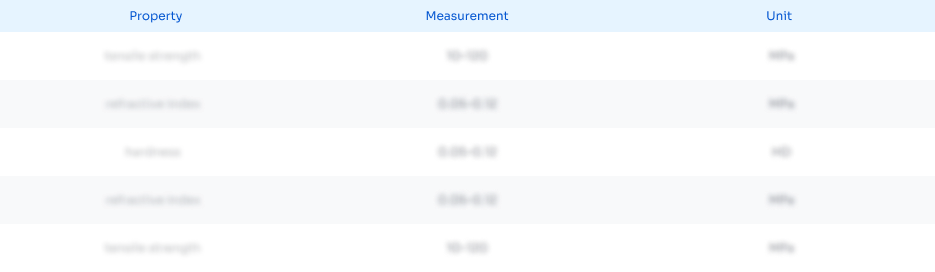
Abstract
Description
Claims
Application Information
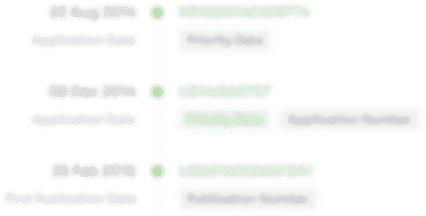
- R&D Engineer
- R&D Manager
- IP Professional
- Industry Leading Data Capabilities
- Powerful AI technology
- Patent DNA Extraction
Browse by: Latest US Patents, China's latest patents, Technical Efficacy Thesaurus, Application Domain, Technology Topic, Popular Technical Reports.
© 2024 PatSnap. All rights reserved.Legal|Privacy policy|Modern Slavery Act Transparency Statement|Sitemap|About US| Contact US: help@patsnap.com