Interest point check-in prediction method fusing deep learning with factorization machine
A technology of factorization and deep learning, applied in forecasting, special data processing applications, instruments, etc., to achieve the effect of reducing blindness
- Summary
- Abstract
- Description
- Claims
- Application Information
AI Technical Summary
Benefits of technology
Problems solved by technology
Method used
Image
Examples
Embodiment Construction
[0032] The preferred embodiments of the present invention will be described in detail below with reference to the accompanying drawings.
[0033] Such as Figure 1-7 As shown, the present invention is a method for predicting check-in of points of interest that integrates deep learning and factorization machines, including the following steps:
[0034] Step S1: Obtain user check-in data, which is divided into continuous feature data and discrete feature data;
[0035] Step S2: Perform embedding processing on the input discrete data, and map discrete features to low-dimensional vectors through a structure similar to local connections;
[0036] Step S3: use a network structure similar to FM to perform sparse processing of discrete data, and learn the implicit second-order relationship between data;
[0037] Step S4: Add a fully connected hidden layer for continuous features (weather, spatial information) for learning, and select an appropriate activation function;
[0038] Ste...
PUM
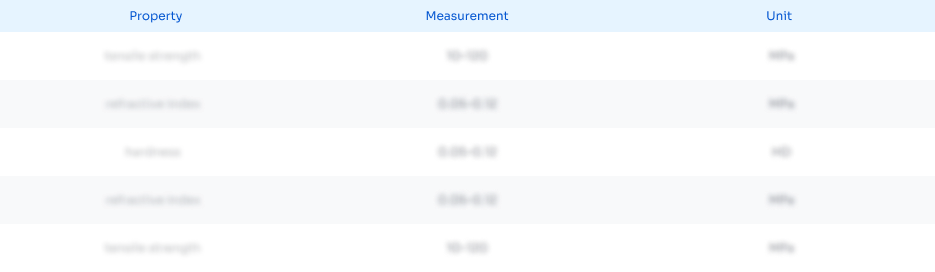
Abstract
Description
Claims
Application Information
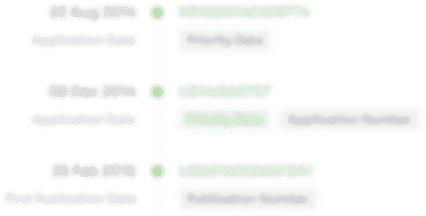
- R&D Engineer
- R&D Manager
- IP Professional
- Industry Leading Data Capabilities
- Powerful AI technology
- Patent DNA Extraction
Browse by: Latest US Patents, China's latest patents, Technical Efficacy Thesaurus, Application Domain, Technology Topic, Popular Technical Reports.
© 2024 PatSnap. All rights reserved.Legal|Privacy policy|Modern Slavery Act Transparency Statement|Sitemap|About US| Contact US: help@patsnap.com