Multi-mode complex activity recognition method based on deep learning model
A technology of deep learning and activity recognition, applied in the field of activity recognition, to achieve the effect of improving accuracy and increasing non-linear capabilities
- Summary
- Abstract
- Description
- Claims
- Application Information
AI Technical Summary
Problems solved by technology
Method used
Image
Examples
Embodiment Construction
[0038] In order to make the object, technical solution and advantages of the present invention clearer, the present invention will be further described in detail below in conjunction with the accompanying drawings and embodiments. It should be understood that the specific embodiments described here are only used to explain the present invention, and do not limit the protection scope of the present invention.
[0039]In order to solve the problems in the background technology, the present invention defines complex activities as a simple semantic sequence of activities, and then extracts sequence features to complete complex activity recognition tasks. Firstly, the time series data of different modalities are divided into three categories according to their attributes; then convolutional sub-networks with different structures are constructed for feature extraction; then the longitudinal splicing layer and convolutional layer are used to fuse the features of different modalities; ...
PUM
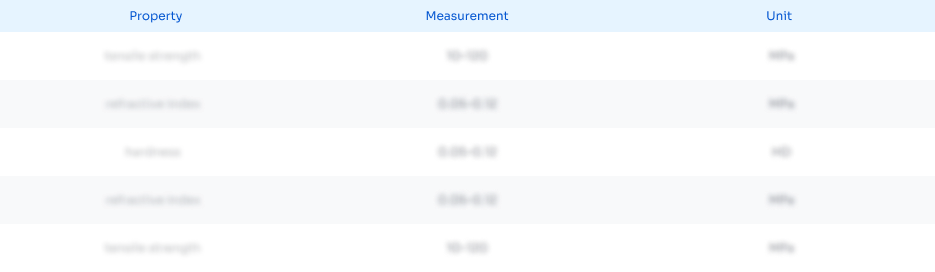
Abstract
Description
Claims
Application Information
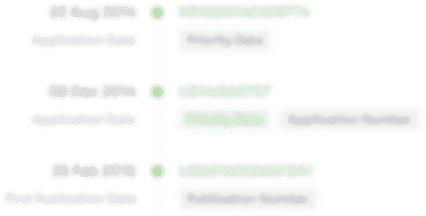
- R&D Engineer
- R&D Manager
- IP Professional
- Industry Leading Data Capabilities
- Powerful AI technology
- Patent DNA Extraction
Browse by: Latest US Patents, China's latest patents, Technical Efficacy Thesaurus, Application Domain, Technology Topic, Popular Technical Reports.
© 2024 PatSnap. All rights reserved.Legal|Privacy policy|Modern Slavery Act Transparency Statement|Sitemap|About US| Contact US: help@patsnap.com