Machine learning method based on weighted depth forest
A machine learning and forest technology, applied in the application field, can solve problems such as error amplification, achieve performance improvement, reduce cascade series, and improve prediction accuracy
- Summary
- Abstract
- Description
- Claims
- Application Information
AI Technical Summary
Problems solved by technology
Method used
Image
Examples
Embodiment Construction
[0050] In order to make the object, technical solution and advantages of the present invention clearer, the present invention will be described in detail below in conjunction with the accompanying drawings and specific embodiments.
[0051] The invention provides a machine learning method based on weighted deep forest. Deep forest includes multi-granularity scanning and cascading forest. Multi-granularity scanning can generate corresponding class vectors by obtaining multiple feature subsets, and then stitch the class vectors into the feature space of the original sample as the input features of the subsequent cascading forest. Cascade forests are used to realize representation learning, including random forests and completely random tree forests, and cascade structures are formed between forests in a hierarchical manner. The structure of a single weighted forest is as Figure 4 As shown, by calculating the corresponding weight of the prediction accuracy of each subtree in ea...
PUM
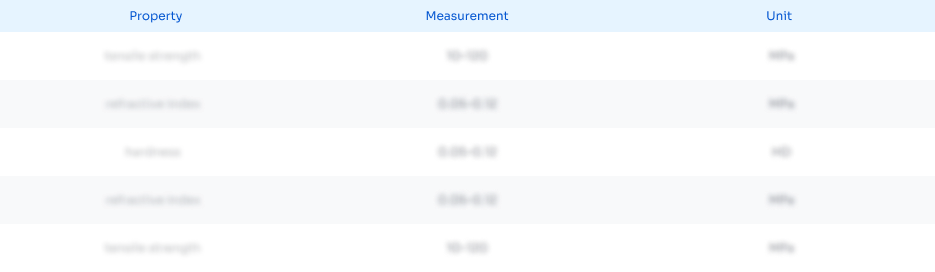
Abstract
Description
Claims
Application Information
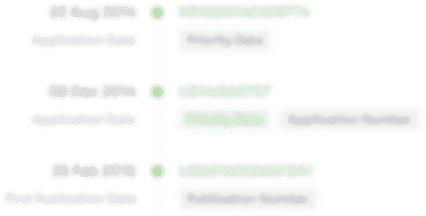
- R&D Engineer
- R&D Manager
- IP Professional
- Industry Leading Data Capabilities
- Powerful AI technology
- Patent DNA Extraction
Browse by: Latest US Patents, China's latest patents, Technical Efficacy Thesaurus, Application Domain, Technology Topic, Popular Technical Reports.
© 2024 PatSnap. All rights reserved.Legal|Privacy policy|Modern Slavery Act Transparency Statement|Sitemap|About US| Contact US: help@patsnap.com