A target detection method based on generative adversarial network
A target detection and target technology, applied in the field of target detection, can solve the problems of complex computing memory requirements, less research on generated data, hardware consumption, etc., to achieve the effects of enriching training data, easy deployment, and improving detection accuracy
Active Publication Date: 2022-07-15
HARBIN ENG UNIV
View PDF2 Cites 0 Cited by
- Summary
- Abstract
- Description
- Claims
- Application Information
AI Technical Summary
Problems solved by technology
Secondly, most of the target detection research at this stage relies on deeper networks to improve accuracy, but this will bring more complex calculations and more memory requirements, consume a lot of hardware, and be difficult to deploy
Generating
Method used
the structure of the environmentally friendly knitted fabric provided by the present invention; figure 2 Flow chart of the yarn wrapping machine for environmentally friendly knitted fabrics and storage devices; image 3 Is the parameter map of the yarn covering machine
View moreImage
Smart Image Click on the blue labels to locate them in the text.
Smart ImageViewing Examples
Examples
Experimental program
Comparison scheme
Effect test
Embodiment 1
[0036] A target detection method based on generative adversarial network, the specific implementation steps are as follows:
[0037] Step 1. Design the generator G to generate various samples according to the category labels, such as figure 2 shown;
the structure of the environmentally friendly knitted fabric provided by the present invention; figure 2 Flow chart of the yarn wrapping machine for environmentally friendly knitted fabrics and storage devices; image 3 Is the parameter map of the yarn covering machine
Login to view more PUM
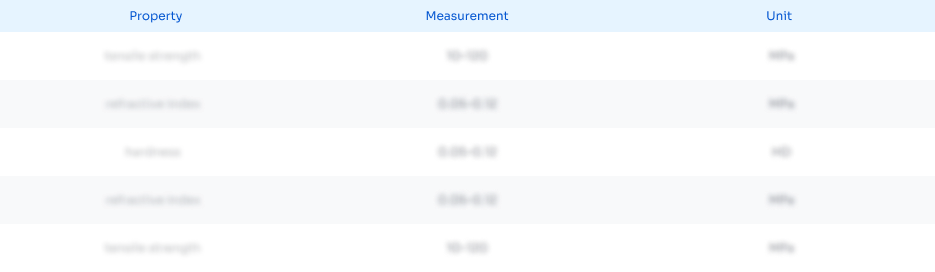
Abstract
The invention provides a target detection method based on a generative confrontation network, which includes designing a generator, generating various samples according to category labels, designing an agent, detecting the data of the generator, providing false true values, and applying the data generated by the agent to Training of target detectors, designing target detectors, judging whether the generated data is conducive to improving the accuracy of target detection, designing adversaries, in the training phase, judging whether the data comes from real data or generated data, the generator and the discriminator are trained alternately, In the testing phase, the data to be detected is directly input into the target detector to obtain the detection result. The combination of the samples generated by the generation network of the invention and the real samples can enrich the training data, improve the detection accuracy, the target detection network provides feedback to the generation network, so that the generated samples are more realistic, and the data generated by the agent is directly applied to the training of the target detector. There is no need to spend a lot of manpower and material resources for labeling, and the present invention has a simple structure and is easy to deploy.
Description
technical field [0001] The invention belongs to the field of target detection methods, in particular to a target detection method based on a generative confrontation network. Background technique [0002] In recent years, deep learning has developed rapidly, and target detection algorithms based on deep learning have achieved good results, but there are still problems to be solved. First, target detection algorithms based on deep learning require a large number of labeled samples, and deep learning is often prone to overfitting on small data sets. According to research, a rough rule of thumb is that supervised deep learning algorithms will generally achieve acceptable performance given about 5,000 labeled samples per class, when a dataset of at least 10 million labeled samples is used for training. will meet or exceed human performance. In addition, the labeling of the dataset will also consume a lot of manpower and material resources. Therefore, how to use a large number...
Claims
the structure of the environmentally friendly knitted fabric provided by the present invention; figure 2 Flow chart of the yarn wrapping machine for environmentally friendly knitted fabrics and storage devices; image 3 Is the parameter map of the yarn covering machine
Login to view more Application Information
Patent Timeline
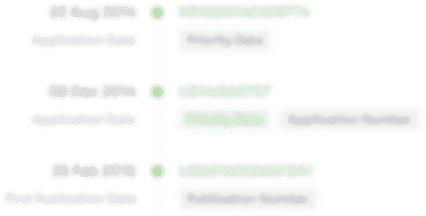
IPC IPC(8): G06V10/764G06V10/774G06V10/80G06V10/82G06K9/62G06N3/04G06N3/08
CPCG06N3/08G06N3/045G06F18/24G06F18/214
Inventor 项学智于泽婷翟明亮吕宁郭鑫立王帅张荣芳张玉琦
Owner HARBIN ENG UNIV
Who we serve
- R&D Engineer
- R&D Manager
- IP Professional
Why Eureka
- Industry Leading Data Capabilities
- Powerful AI technology
- Patent DNA Extraction
Social media
Try Eureka
Browse by: Latest US Patents, China's latest patents, Technical Efficacy Thesaurus, Application Domain, Technology Topic.
© 2024 PatSnap. All rights reserved.Legal|Privacy policy|Modern Slavery Act Transparency Statement|Sitemap