An enterprise finance crisis prediction method based on maximized variance projection subspace multi-core learning
A technology of multi-core learning and forecasting method, applied in the field of enterprise financial crisis, can solve the problems of high difficulty of multi-core forecasting and low forecasting accuracy, and achieve the effect of reducing existing complex multi-core forecasting problems, improving forecasting accuracy and improving forecasting accuracy.
- Summary
- Abstract
- Description
- Claims
- Application Information
AI Technical Summary
Problems solved by technology
Method used
Image
Examples
specific Embodiment approach 1
[0030] Specific implementation mode 1: The specific process of the enterprise financial crisis prediction method based on the maximum variance projection subspace multi-core learning in this implementation mode is as follows:
[0031] Step 1: Select the financial indicators of listed companies;
[0032] Financial indicators in financial crisis prediction mainly come from six aspects: profitability, solvency, development ability, asset management, cash flow, and financial flexibility. normally,
[0033] For a sample X of listed companies, sample X contains t financial indicator features (financial indicators), namely
[0034] in Represents the value corresponding to the λth financial indicator feature in sample X, and the superscript F represents the feature (the superscript F represents the feature feature, and the superscript used as a mark is represented by an uppercase English letter and separated by an underscore _, the same as below ), t is the total number of finan...
specific Embodiment approach 2
[0050] Specific embodiment 2: The difference between this embodiment and specific embodiment 1 is that in the step 1, usually, the number of financial indicators used to characterize the financial status of listed companies in the financial crisis prediction is about 20-30, and usually the characteristics of financial indicators are mainly Including: net profit margin, main business profit margin, return on total assets, return on net assets, non-recurring profit and loss ratio, price-earnings ratio, price-to-book ratio, current ratio, quick ratio, asset-liability ratio, equity ratio, interest protection Multiples, tangible net debt ratio, operating cash flow ratio, main business income growth rate, net profit growth rate, net asset growth rate, total asset growth rate, earnings per share growth rate, operating income per share growth rate, net per share Asset Growth Rate, EBITDA Growth Rate, Accounts Receivable Turnover Ratio, Inventory Turnover Ratio, Current Assets Turnover ...
specific Embodiment approach 3
[0052] Specific embodiment three: the difference between this embodiment and specific embodiment one or two is that in step 3, data normalization processing is performed on the total sample set of ST samples and non-ST samples in step 2;
[0053] The data normalization process adopts the following method:
[0054]
[0055] in Represents the normalized sample features (the superscript N stands for normalization normalization), X n for S ALL A sample of companies in (X n ∈ S ALL ); for X n The value corresponding to the mth feature, t is the total number of financial index features, m=1,2...,t.
[0056] Other steps and parameters are the same as those in Embodiment 1 or Embodiment 2.
PUM
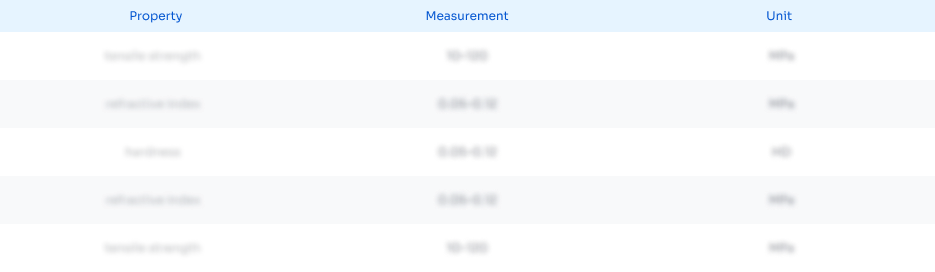
Abstract
Description
Claims
Application Information
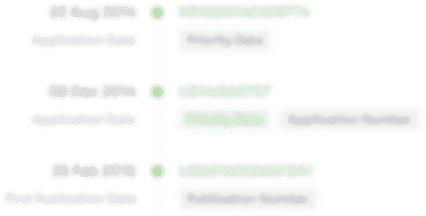
- R&D Engineer
- R&D Manager
- IP Professional
- Industry Leading Data Capabilities
- Powerful AI technology
- Patent DNA Extraction
Browse by: Latest US Patents, China's latest patents, Technical Efficacy Thesaurus, Application Domain, Technology Topic.
© 2024 PatSnap. All rights reserved.Legal|Privacy policy|Modern Slavery Act Transparency Statement|Sitemap