Image processing method and device and computer storage medium
An image processing and target image technology, applied in the field of image processing, can solve the problems of semantic segmentation and segmentation accuracy reduction
- Summary
- Abstract
- Description
- Claims
- Application Information
AI Technical Summary
Problems solved by technology
Method used
Image
Examples
Embodiment 1
[0062] figure 1 is a block diagram of the hardware configuration of the image processing system 100 according to the embodiment of the present invention.
[0063] Such as figure 1 As shown, the image processing system 100 includes an image acquisition device 1000 and an image processing device 2000 .
[0064] The image acquisition device 1000 is configured to acquire a 2D image to be detected, and provide the acquired 2D image to be detected to the image processing device 2000 .
[0065] The image acquisition device 1000 may be any imaging device capable of taking pictures to obtain a target image, that is, an image to be semantically segmented, such as a camera. Alternatively, means capable of acquiring an image of an object from an imaging device.
[0066] The image processing apparatus 2000 may be any electronic device, such as a PC, a notebook computer, a server, and the like.
[0067] In this example, refer to figure 1 As shown, the image processing device 2000 may i...
Embodiment 2
[0074] An image processing method provided in this embodiment, such as figure 2 As shown, including the following steps S201-S204:
[0075] S201. Extract feature map groups of different scales of the target image, wherein each feature map in the same feature map group has different scales.
[0076] In this embodiment, the aforementioned target image is an image to be semantically segmented. This image is usually an RGB image.
[0077] In one embodiment, the above S201 is implemented through the following S2011 and S2012:
[0078] S2011. Input the target image into the convolutional neural network to obtain a first feature map of the target image for each scale in different scales.
[0079] In the first embodiment, the specific implementation of the above S2011 may be as follows: input the target image into the convolutional neural network, use the convolutional neural network to perform image feature extraction on the target image, and obtain multiple feature maps with cor...
Embodiment 3
[0152] Such as Figure 6 As shown, the embodiment of the present invention also provides an image processing apparatus 6000 . The image processing device 6000 includes: an extraction module 6100 , a fusion module 6200 , and a segmentation module 6300 . in:
[0153] The extraction module 6100 is used to extract feature map groups of different scales of the target image, wherein each feature map in the same feature map group has the same scale;
[0154] The fusion module 6200 is configured to perform feature fusion on the same scale for all the feature map groups to obtain the corresponding first fusion feature map;
[0155] The fusion module 6200 is further configured to enlarge each of the first fusion feature maps to the largest scale among the different scales, and perform feature fusion at the same scale on all the enlarged first fusion feature maps to obtain the second fusion feature map;
[0156] The segmentation module 6300 is configured to obtain a semantic segmenta...
PUM
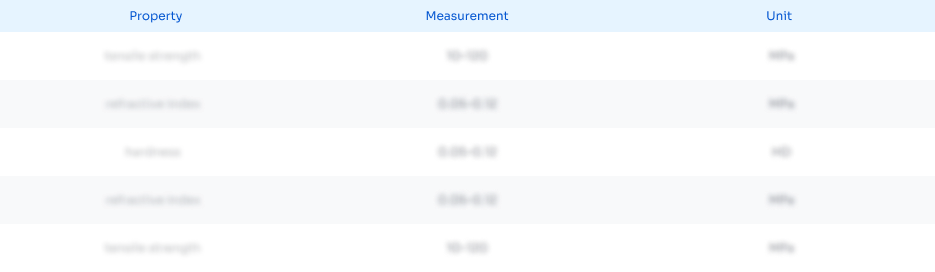
Abstract
Description
Claims
Application Information
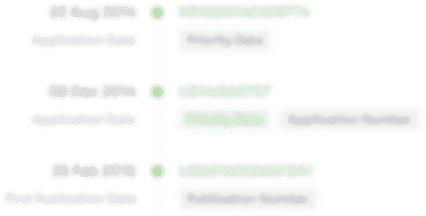
- R&D Engineer
- R&D Manager
- IP Professional
- Industry Leading Data Capabilities
- Powerful AI technology
- Patent DNA Extraction
Browse by: Latest US Patents, China's latest patents, Technical Efficacy Thesaurus, Application Domain, Technology Topic, Popular Technical Reports.
© 2024 PatSnap. All rights reserved.Legal|Privacy policy|Modern Slavery Act Transparency Statement|Sitemap|About US| Contact US: help@patsnap.com