A Binocular Depth Estimation Method for Driving Scenes Overcoming Occlusion Effect
A driving scene, depth estimation technology, applied in the field of machine vision and autonomous driving, can solve the problems of poor accuracy, decreased global accuracy, consistent views, etc., to deal with occlusion effects, enhance robustness, and overcome occlusion effects.
- Summary
- Abstract
- Description
- Claims
- Application Information
AI Technical Summary
Problems solved by technology
Method used
Image
Examples
Embodiment Construction
[0053] In order to clarify the purpose, technical solutions and features of the embodiments of the present invention, the technical solutions in the embodiments of the present invention will be clearly and completely described below with reference to the accompanying drawings of the present invention. Obviously, the described embodiments are part, but not all, of the method of implementing the present invention. All other implementation manners obtained by those of ordinary skill in the art without creative efforts fall within the protection scope of the present invention. Accordingly, the following descriptions of messages provided in the accompanying drawings of the present invention are not intended to limit the scope of protection of the claimed invention, but merely represent selected embodiments of the invention. Based on the embodiments of the present invention, all other embodiments obtained by those of ordinary skill in the art without creative efforts shall fall with...
PUM
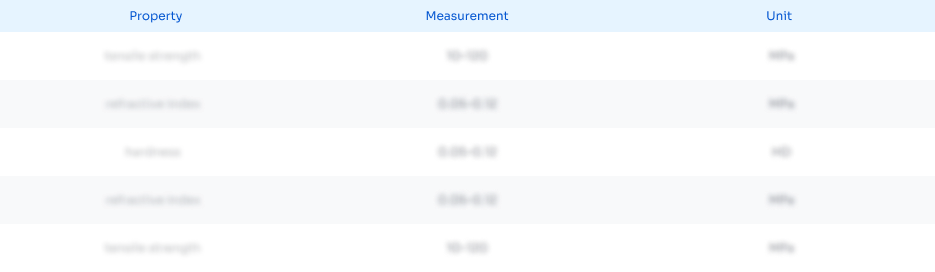
Abstract
Description
Claims
Application Information
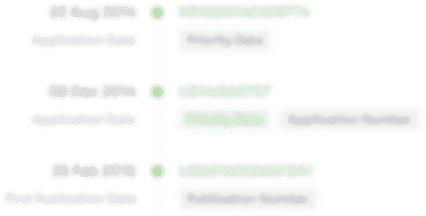
- R&D
- Intellectual Property
- Life Sciences
- Materials
- Tech Scout
- Unparalleled Data Quality
- Higher Quality Content
- 60% Fewer Hallucinations
Browse by: Latest US Patents, China's latest patents, Technical Efficacy Thesaurus, Application Domain, Technology Topic, Popular Technical Reports.
© 2025 PatSnap. All rights reserved.Legal|Privacy policy|Modern Slavery Act Transparency Statement|Sitemap|About US| Contact US: help@patsnap.com