Unsupervised cross-domain pedestrian re-identification method based on progressive enhanced self-learning
A pedestrian re-identification and progressive enhancement technology, applied in the field of image processing, can solve problems such as performance deviation, huge manpower and time costs, poor effect, etc., to achieve the effect of improving accuracy and recall rate, improving representation ability, and improving convergence ability
- Summary
- Abstract
- Description
- Claims
- Application Information
AI Technical Summary
Problems solved by technology
Method used
Image
Examples
Embodiment Construction
[0035] The progressively enhanced self-learning unsupervised cross-domain pedestrian re-identification method provided by the present invention has the following overall idea:
[0036] First, the initial network model trained on the labeled original domain dataset is given, and then the initial features of the entire unlabeled target domain training dataset are extracted (as the initial input of the entire method), and the cosine similarity between any pair of features is calculated degree, sort the cosine similarity from high to low to get the similarity score matrix, and then use the HDBSCAN clustering algorithm to cluster on the similarity score matrix to generate a subset of target domain training data with pseudo-class labels, and here Use the triplet loss function to retrain the network model on the target domain training data subset with pseudo-class labels, optimize the local features, and then extract the features of the current network model on the target domain train...
PUM
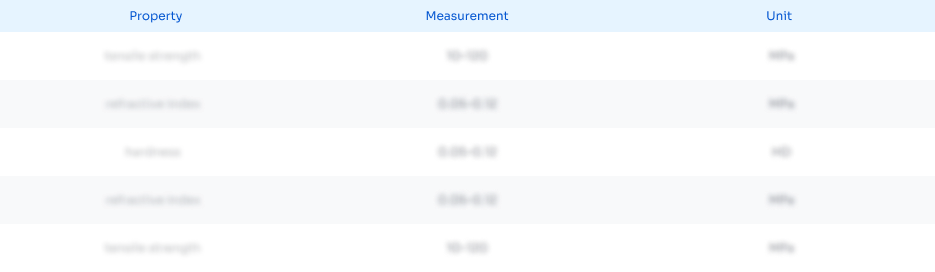
Abstract
Description
Claims
Application Information
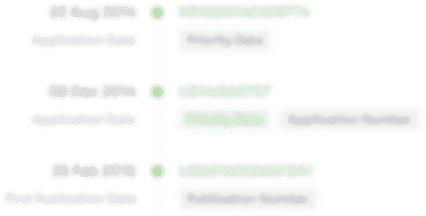
- R&D Engineer
- R&D Manager
- IP Professional
- Industry Leading Data Capabilities
- Powerful AI technology
- Patent DNA Extraction
Browse by: Latest US Patents, China's latest patents, Technical Efficacy Thesaurus, Application Domain, Technology Topic, Popular Technical Reports.
© 2024 PatSnap. All rights reserved.Legal|Privacy policy|Modern Slavery Act Transparency Statement|Sitemap|About US| Contact US: help@patsnap.com