Dynamic predictive machine learning type air conditioner energy-saving control method
A technology of machine learning and dynamic prediction, applied in the direction of mechanical equipment, etc., can solve the problems that the central air-conditioning system is difficult to obtain a good and stable energy-saving control effect, affects the timeliness and rapidity of control, and the limitation of a single energy-saving adjustment strategy. The effects of intelligent management and stable operation, timeliness and rapidity, improvement of prediction accuracy and parameter optimization performance
- Summary
- Abstract
- Description
- Claims
- Application Information
AI Technical Summary
Benefits of technology
Problems solved by technology
Method used
Image
Examples
Embodiment Construction
[0018] In order to make the objectives, technical solutions, and advantages of the present invention clearer and clearer, the content of the present invention will be further described in detail below in conjunction with the accompanying drawings and specific embodiments. It can be understood that the specific embodiments described here are only used to explain the present invention, but not to limit the present invention. In addition, it should be noted that, for ease of description, the drawings only show a part but not all of the content related to the present invention.
[0019] Such as figure 1 As shown, this embodiment proposes a dynamic predictive machine learning type air conditioning energy-saving control method, which includes the following steps S1-S5:
[0020] S1. Collect hourly environmental parameters: including dry bulb temperature, relative humidity, and total horizontal solar radiation; record building hourly usage parameters: including occupancy rate, hour label a...
PUM
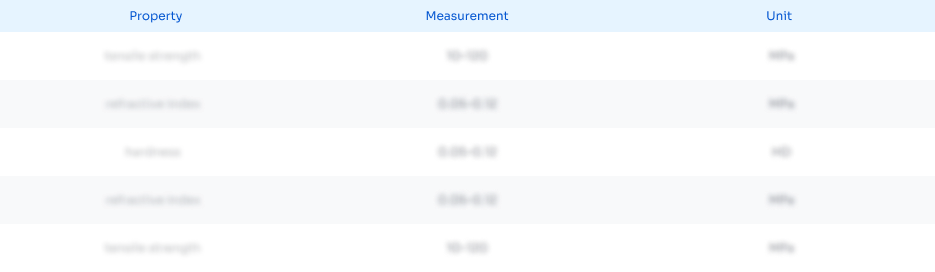
Abstract
Description
Claims
Application Information
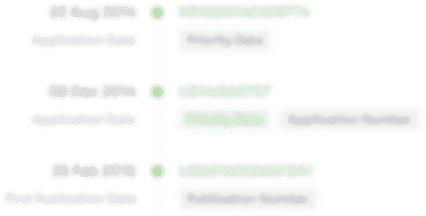
- R&D Engineer
- R&D Manager
- IP Professional
- Industry Leading Data Capabilities
- Powerful AI technology
- Patent DNA Extraction
Browse by: Latest US Patents, China's latest patents, Technical Efficacy Thesaurus, Application Domain, Technology Topic.
© 2024 PatSnap. All rights reserved.Legal|Privacy policy|Modern Slavery Act Transparency Statement|Sitemap