Distributed learning privacy protection method based on differential privacy
A privacy protection and differential privacy technology, applied in digital data protection, character and pattern recognition, instruments, etc.
- Summary
- Abstract
- Description
- Claims
- Application Information
AI Technical Summary
Problems solved by technology
Method used
Image
Examples
Embodiment Construction
[0044] In this embodiment, a distributed learning privacy protection method based on differential privacy is applied to a weighted network graph G(V, E) composed of n user nodes, where V represents n user nodes in the weighted network graph G A collection of ; E is the relationship edge connected between user nodes; record W∈R n×n is the symmetric non-negative weight matrix associated with the weighted network graph G;
[0045] Denote i, j as the serial numbers of any two user nodes in the weighted network graph G, and define W ij is the weight of the relationship edge (i,j)∈E between the i-th user node and the j-th user node, and the weight W ij Meet: W ij ∈[0,1],W ij =W ji , when W ij= 0, it means that the relationship edge (i, j) between the i-th user node and the j-th user node is not connected; when W ij When ≠0, it means that the relationship edge (i, j) between the i-th user node and the j-th user node is connected.
[0046] The i-th user node has a local data di...
PUM
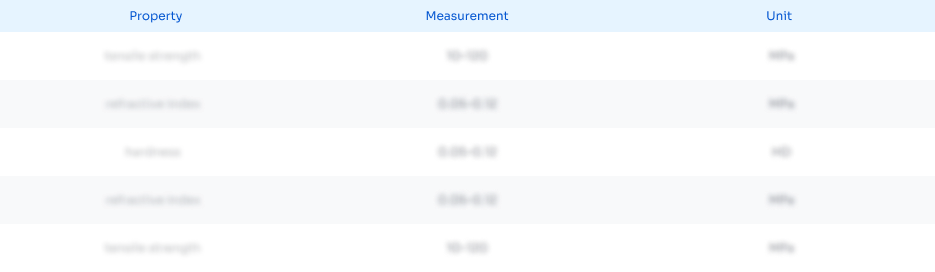
Abstract
Description
Claims
Application Information
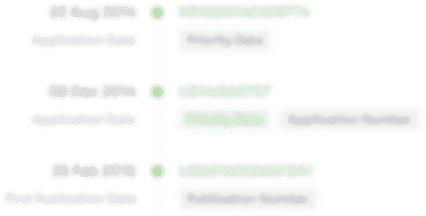
- R&D Engineer
- R&D Manager
- IP Professional
- Industry Leading Data Capabilities
- Powerful AI technology
- Patent DNA Extraction
Browse by: Latest US Patents, China's latest patents, Technical Efficacy Thesaurus, Application Domain, Technology Topic, Popular Technical Reports.
© 2024 PatSnap. All rights reserved.Legal|Privacy policy|Modern Slavery Act Transparency Statement|Sitemap|About US| Contact US: help@patsnap.com