Multi-objective multi-modal particle swarm optimization method based on Bayesian adaptive resonance
A multi-modal particle and optimization method technology, applied in the field of optimization algorithms, can solve problems such as uncertainty and problem dependence
- Summary
- Abstract
- Description
- Claims
- Application Information
AI Technical Summary
Problems solved by technology
Method used
Image
Examples
Embodiment Construction
[0091] The following will clearly and completely describe the technical solutions in the embodiments of the present invention with reference to the accompanying drawings in the embodiments of the present invention. Obviously, the described embodiments are only some, not all, embodiments of the present invention. Based on the embodiments of the present invention, all other embodiments obtained by persons of ordinary skill in the art without making creative efforts belong to the protection scope of the present invention.
[0092] The embodiment of the present invention discloses a multi-objective multi-modal particle swarm optimization method based on Bayesian adaptive resonance, the method steps are as follows figure 1 As shown, the specific process of the algorithm is as follows figure 2 As shown, the specific content is as follows:
[0093] Step 1: Determine the relevant parameters of the particle swarm and initialize the particle swarm.
[0094] The relevant parameters he...
PUM
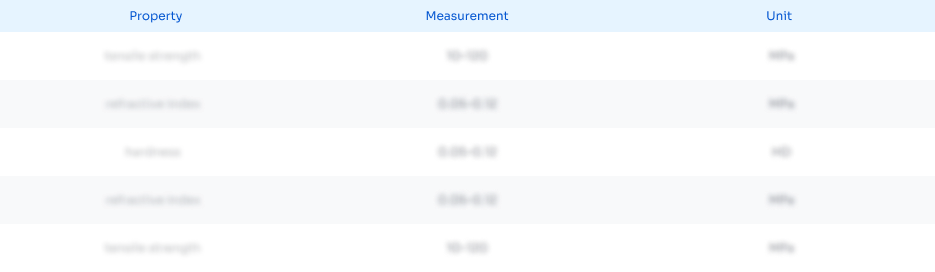
Abstract
Description
Claims
Application Information
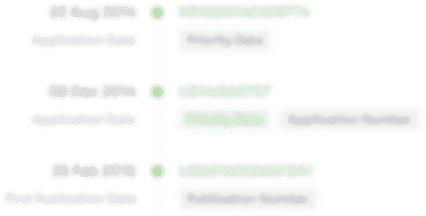
- Generate Ideas
- Intellectual Property
- Life Sciences
- Materials
- Tech Scout
- Unparalleled Data Quality
- Higher Quality Content
- 60% Fewer Hallucinations
Browse by: Latest US Patents, China's latest patents, Technical Efficacy Thesaurus, Application Domain, Technology Topic, Popular Technical Reports.
© 2025 PatSnap. All rights reserved.Legal|Privacy policy|Modern Slavery Act Transparency Statement|Sitemap|About US| Contact US: help@patsnap.com