Semi-supervising method without consistency constraint and mobile terminal
A semi-supervised and consistent technology, applied in the field of deep learning, can solve problems such as poor effect and low model generalization ability, and achieve the effect of improving classification effect and generalization ability
- Summary
- Abstract
- Description
- Claims
- Application Information
AI Technical Summary
Problems solved by technology
Method used
Image
Examples
Embodiment Construction
[0020] The following will clearly and completely describe the technical solutions in the embodiments of the application with reference to the drawings in the embodiments of the application. Apparently, the described embodiments are only some of the embodiments of the application, not all of them. Based on the embodiments in this application, all other embodiments obtained by persons of ordinary skill in the art without making creative efforts belong to the scope of protection of this application.
[0021] It should be noted that if there is a directional indication (such as up, down, left, right, front, back, etc.) in the embodiment of the present application, the directional indication is only used to explain the position in a certain posture ( As shown in the accompanying drawings), if the specific posture changes, the directional indication will also change accordingly.
[0022] In addition, if there are descriptions involving "first", "second", etc. in the embodiments of t...
PUM
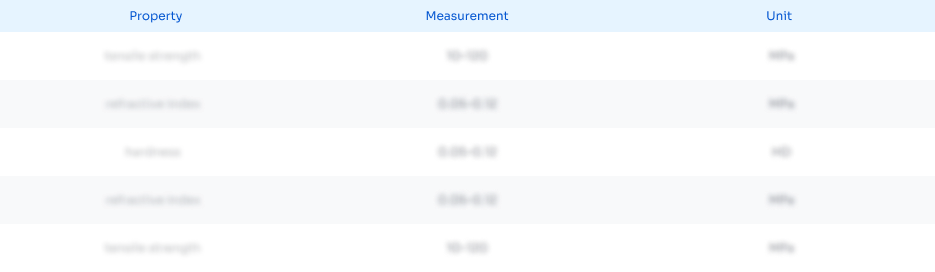
Abstract
Description
Claims
Application Information
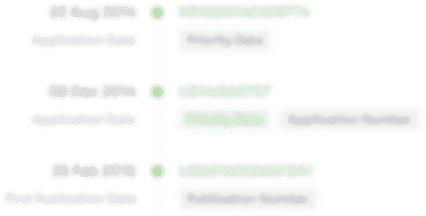
- R&D Engineer
- R&D Manager
- IP Professional
- Industry Leading Data Capabilities
- Powerful AI technology
- Patent DNA Extraction
Browse by: Latest US Patents, China's latest patents, Technical Efficacy Thesaurus, Application Domain, Technology Topic, Popular Technical Reports.
© 2024 PatSnap. All rights reserved.Legal|Privacy policy|Modern Slavery Act Transparency Statement|Sitemap|About US| Contact US: help@patsnap.com