Vehicle detection method based on improved YOLOv3
A vehicle detection and vehicle technology, which is applied in the field of deep learning and intelligent vehicle road detection, can solve the problems of increased missed detection rate, poor detection effect and accuracy, and inability to obtain detection results, etc.
- Summary
- Abstract
- Description
- Claims
- Application Information
AI Technical Summary
Problems solved by technology
Method used
Image
Examples
Embodiment Construction
[0086] Below in conjunction with accompanying drawing, concrete example of the present invention is described in further detail:
[0087] The present invention proposes a vehicle detection method based on improved YOLOv3, the overall flow of the method is as follows figure 1 As shown, it specifically includes the following steps:
[0088] (1) Build as figure 2 The shown YOLO-TN network redesigns the convolutional neural network structure between the Darknet layer and the three yolo layers, and draws on the idea of TridentNet weight sharing to design a network with three parallel branches, each of which joins For dilated convolution, the dilation rate of the three branch networks is set to 3, 2, and 1 respectively so that each branch has a different receptive field (receptive field), and a scale-aware training scheme is designed to ensure that each branch can Train on targets that match the receptive field of that branch. Except that the dilation rate is different, the co...
PUM
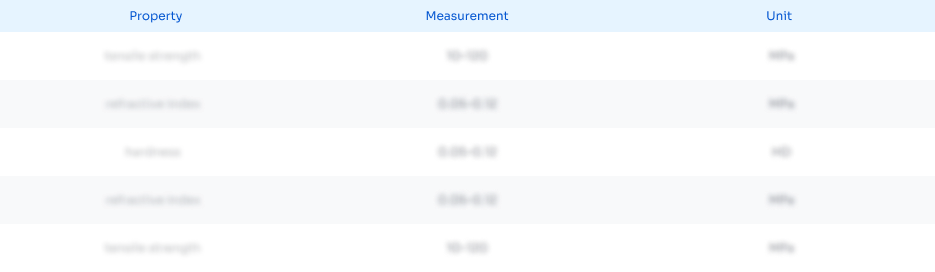
Abstract
Description
Claims
Application Information
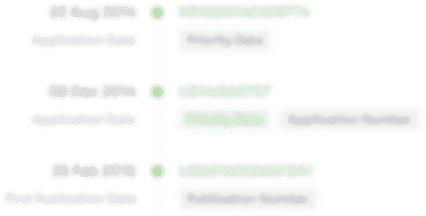
- R&D Engineer
- R&D Manager
- IP Professional
- Industry Leading Data Capabilities
- Powerful AI technology
- Patent DNA Extraction
Browse by: Latest US Patents, China's latest patents, Technical Efficacy Thesaurus, Application Domain, Technology Topic, Popular Technical Reports.
© 2024 PatSnap. All rights reserved.Legal|Privacy policy|Modern Slavery Act Transparency Statement|Sitemap|About US| Contact US: help@patsnap.com