Cross-working-condition fault diagnosis method based on open set joint transfer learning
A technology of fault diagnosis and transfer learning, applied in neural learning methods, instruments, biological neural network models, etc., can solve problems such as failure to guarantee the same failure modes of failure types and failure to achieve effective migration.
- Summary
- Abstract
- Description
- Claims
- Application Information
AI Technical Summary
Problems solved by technology
Method used
Image
Examples
specific Embodiment
[0102] In order to verify the effectiveness of the cross-working condition fault diagnosis method based on open set joint migration proposed by the present invention, the industrial gearbox dataset released in the 2009 PHM Data Challenge was used for case verification. The gearbox data set is collected from an industrial two-stage reduction gearbox, and an acceleration sensor is arranged at the input end of the gearbox to collect vibration data signals of the gearbox, such as Figure 5 shown. The number of teeth of the input shaft gear is 32, the number of teeth of the intermediate shaft gear is 48, and the first-stage reduction ratio is 1.5; the number of teeth of the output shaft gear is 80, and the second-stage reduction ratio is 1.667.
PUM
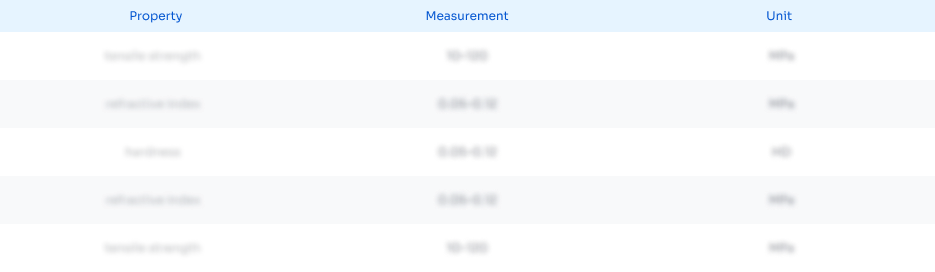
Abstract
Description
Claims
Application Information
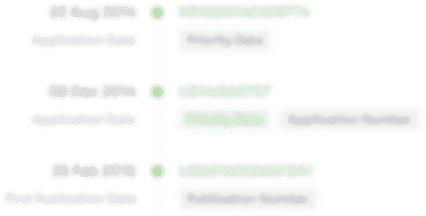
- R&D Engineer
- R&D Manager
- IP Professional
- Industry Leading Data Capabilities
- Powerful AI technology
- Patent DNA Extraction
Browse by: Latest US Patents, China's latest patents, Technical Efficacy Thesaurus, Application Domain, Technology Topic, Popular Technical Reports.
© 2024 PatSnap. All rights reserved.Legal|Privacy policy|Modern Slavery Act Transparency Statement|Sitemap|About US| Contact US: help@patsnap.com