Semi-supervised image semantic segmentation method and device based on self-supervised low-rank network
A semantic segmentation and semi-supervised technology, applied in image analysis, image enhancement, image data processing, etc., can solve the problems of gradient flow backpropagation, increasing error imitation and accumulation, etc., to enhance consistency, solve ambiguity or The effect of misprediction
- Summary
- Abstract
- Description
- Claims
- Application Information
AI Technical Summary
Problems solved by technology
Method used
Image
Examples
Embodiment 1
[0099] To evaluate the effectiveness of SLRNet, extensive experiments are conducted on the Pascal VOC 2012 dataset, which is a widely used WSSS evaluation benchmark. In addition, an enhanced training set is constructed by adding annotations. There are a total of 10582 images in the dataset for training and 1449 images for validation.
[0100] 1. Model selection and use
[0101] 1. Cross-view self-supervision framework
[0102] A cross-view self-supervision framework is proposed, and by combining the LR low-rank module, the compounding effect caused by the self-supervision error of the single-level WSSS model is effectively alleviated. SLRNet simultaneously predicts multiple segmentation templates for multiple augmented views of an image, and then merges to generate accurate pseudo-labels as cross-view self-supervision. The supervision of cross-views helps to utilize the supplementary information from various augmented views to strengthen the consistency of predictions.
[...
Embodiment 2
[0123] In order to verify the superiority of the method and the effectiveness of each module, a large number of ablation experiments were carried out in the embodiment of the present invention, as described below for details:
[0124] To understand the impact of individual data augmentation on weakly supervised segmentation, several geometric and appearance augmentation modalities are considered. In addition, more attention is paid to reversible and differentiable geometric transformations, such as scaling and flipping, etc.
[0125] First, randomly crop the image to a size of 321×321. Then, apply target transformations to different branches. The composition of three transformations is studied: fixed-rate rescaling, random horizontal flipping, and random color distortions (such as brightness, contrast, saturation, and hue). Under supervised settings, strong color distortion does not improve or even hurt performance. So, for Brightness, Contrast, and Saturation, set the Maxi...
PUM
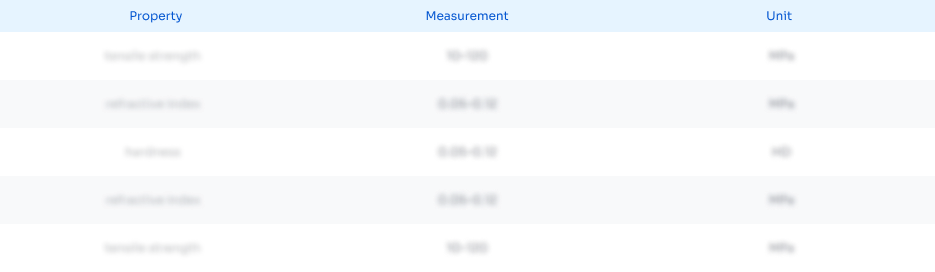
Abstract
Description
Claims
Application Information
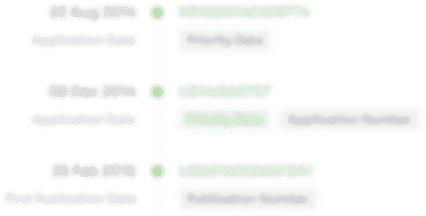
- R&D Engineer
- R&D Manager
- IP Professional
- Industry Leading Data Capabilities
- Powerful AI technology
- Patent DNA Extraction
Browse by: Latest US Patents, China's latest patents, Technical Efficacy Thesaurus, Application Domain, Technology Topic.
© 2024 PatSnap. All rights reserved.Legal|Privacy policy|Modern Slavery Act Transparency Statement|Sitemap