Federal learning-based model parameter updating method, device and equipment
A model parameter and federated technology, applied in the field of data processing, can solve problems such as small amount of data, poor data quality, and poor update accuracy of model parameters
- Summary
- Abstract
- Description
- Claims
- Application Information
AI Technical Summary
Problems solved by technology
Method used
Image
Examples
Embodiment 1
[0034] like Figure 1A and 1B As shown, the embodiment of this specification provides a method for updating model parameters based on federated learning. The execution body of the method may be a federated learning client, and the federated learning client may be a server or a terminal device, wherein the server may be an independent server , can also be a server cluster composed of multiple servers, and the terminal equipment can be equipment such as personal computers, or mobile terminal equipment such as mobile phones and tablet computers. The method specifically may include the following steps:
[0035] In S102, a model parameter update instruction for the target graph neural network model issued by the federated learning server is received.
[0036] Wherein, the model parameter update command may carry the first shared parameter, and the first shared parameter may be the model parameter obtained in the previous model parameter update cycle stored by the federated learnin...
Embodiment 2
[0053] like Figure 4 As shown, the embodiment of this specification provides a method for updating model parameters based on federated learning. The execution body of the method may be a federated learning client, and the federated learning client may be a server or a terminal device, wherein the server may be an independent server , can also be a server cluster composed of multiple servers, and the terminal equipment can be equipment such as personal computers, or mobile terminal equipment such as mobile phones and tablet computers. The method specifically may include the following steps:
[0054] In S402, a model parameter update instruction for the target graph neural network model issued by the federated learning server is received.
[0055] For the specific processing procedure of the above S402, reference may be made to the related content of S102 in the above Embodiment 1, which will not be repeated here.
[0056] In S404, based on the preset neighborhood radius and ...
Embodiment 3
[0092] like Figure 6A and Figure 6B As shown, the embodiment of this specification provides a model parameter update method based on federated learning, the execution subject of the method may be a federated learning server, and the federated learning server may be a server or a terminal device, wherein the server may be an independent server , can also be a server cluster composed of multiple servers, and the terminal equipment can be equipment such as personal computers, or mobile terminal equipment such as mobile phones and tablet computers. The method specifically may include the following steps:
[0093] In S602, a model parameter update instruction for the target graph neural network model is sent to multiple federated learning clients.
[0094] Wherein, the model parameter update instruction may carry the first shared parameter, and the target graph neural network model is a common model of multiple federated learning clients.
[0095] In S604, the second shared pa...
PUM
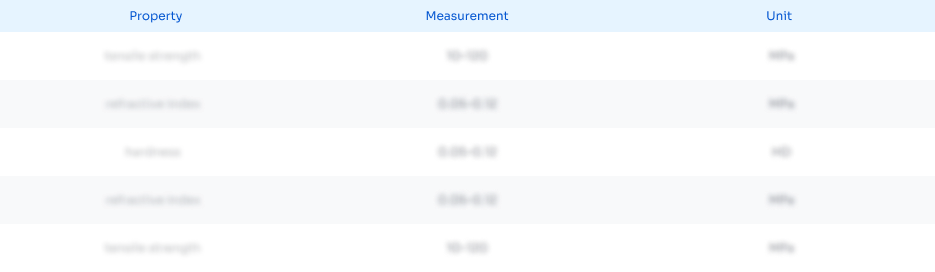
Abstract
Description
Claims
Application Information
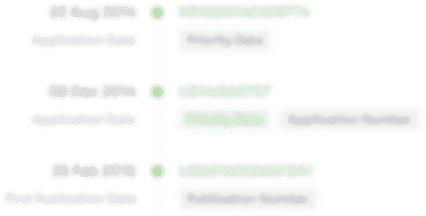
- R&D
- Intellectual Property
- Life Sciences
- Materials
- Tech Scout
- Unparalleled Data Quality
- Higher Quality Content
- 60% Fewer Hallucinations
Browse by: Latest US Patents, China's latest patents, Technical Efficacy Thesaurus, Application Domain, Technology Topic, Popular Technical Reports.
© 2025 PatSnap. All rights reserved.Legal|Privacy policy|Modern Slavery Act Transparency Statement|Sitemap|About US| Contact US: help@patsnap.com