Systems and methods for deriving and optimizing classifiers from multiple datasets
A technique for training data sets, computer systems, applied in the field of systems and methods for deriving and optimizing classifiers from multiple data sets, capable of solving problems such as poor model performance
- Summary
- Abstract
- Description
- Claims
- Application Information
AI Technical Summary
Problems solved by technology
Method used
Image
Examples
Embodiment 1
[0164] Systematic search and inclusion criteria for gene expression studies in clinical infections
[0165] IMX training data meeting defined inclusion criteria for clinical infection studies were obtained from the NCBI GEO (www.ncbi.nlm.nih.gov / geo / ) and EMBL-EBI ArrayExpress (www.ebi.ac.uk / arrayexpress) databases set. Specifically, inclusion criteria included patients in the study who 1) had to be physician-adjudicated for the presence and type of infection (eg, strictly bacterial infection, strictly viral infection, or noninfectious inflammatory disease), and 2) had previously identified Gene expression measurements of 29 diagnostic markers (Sweeney et al., 2015, Sci Transl Med 7(287), pp.287ra71; Sweeney et al, 2016, Sci Transl Med 8(346), pp.346ra91; and Sweeney et al. ., 2018, Nature Communications 9, p.694), 3) over 18 years of age, 4) have been seen in a hospital setting (e.g. emergency department, intensive care), 5) have a community or hospital acquired infection, a...
Embodiment 2
[0167] Normalization of expression data and COCONUT co-normalization
[0168] Normalization was then performed within each study, using one of two methods depending on the platform. For Affymetrix arrays, use Robust Multi-array Average (RMA) (Irizarry et al., 2003, Biostatistics, 4(2):249-64) or gcRMA (Wu et al., 2004, Journal of the American Statistical Association, 99: 909–17) to normalize expression data. Expression data from other platforms were normalized using exponential convolution methods for background correction followed by quantile normalization.
[0169] After normalizing the raw expression data, the COCONUT algorithm (Sweeney et al., 2016, Sci Transl Med 8(346), pp.346ra91; and Abouelhoda et al., 2008, BMC Bioinformatics9, p.476) were used to total Normalize these measurements and ensure they are comparable across studies. Based on the empirical Bayesian batch correction method of ComBat (Johnson et al., 2007, Biostatistics, 8, pp.118-127), COCONUT calculates ...
Embodiment 3
[0171] Developing a sepsis classifier with machine learning
[0172] To develop a sepsis classifier, a machine learning approach was employed. The method involves specifying candidate models, evaluating the performance of different classifiers using training data and specified performance statistics, and then selecting the best performing model to evaluate on independent data.
[0173]In this case, model refers to machine learning algorithms such as logistic regression, neural networks, decision trees, etc. (similar to models used in statistics). Similarly, in this case the main classifier refers to a model with fixed (locked) parameters (weights) and thresholds, which is ready to be applied to previously unseen samples. Classifiers use two types of parameters: weights learned by a core learning algorithm (such as XGBoost), and additional user-supplied parameters that are input to the core learner. These additional parameters are called hyperparameters. Classifier developme...
PUM
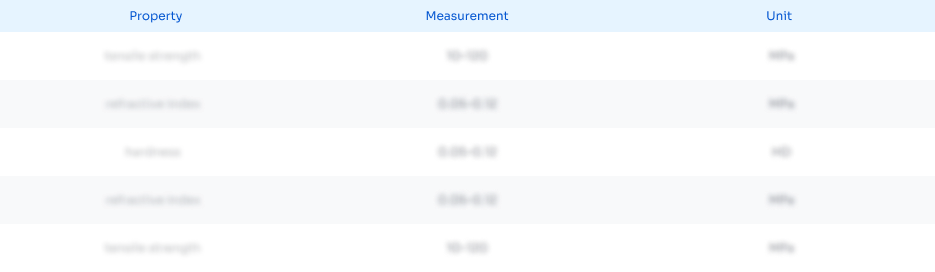
Abstract
Description
Claims
Application Information
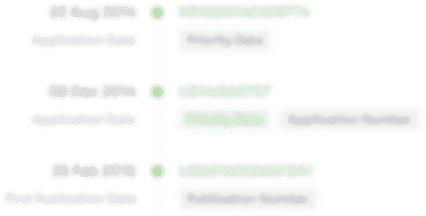
- R&D Engineer
- R&D Manager
- IP Professional
- Industry Leading Data Capabilities
- Powerful AI technology
- Patent DNA Extraction
Browse by: Latest US Patents, China's latest patents, Technical Efficacy Thesaurus, Application Domain, Technology Topic.
© 2024 PatSnap. All rights reserved.Legal|Privacy policy|Modern Slavery Act Transparency Statement|Sitemap