Scratch detection method based on deep convolutional neural network and image segmentation
A neural network and deep convolution technology, applied in the field of automatic defect detection and precision measurement, can solve problems such as difficulty in meeting the requirements of scratch pixel segmentation accuracy, interference, and ignoring recognition, avoiding background information interference, high precision, The effect of fast detection speed
- Summary
- Abstract
- Description
- Claims
- Application Information
AI Technical Summary
Problems solved by technology
Method used
Image
Examples
Embodiment Construction
[0068] Specific embodiments of the present invention will be described in detail below in conjunction with the accompanying drawings.
[0069] Such as figure 1 As shown, the present invention is based on the scratch detection method of deep convolutional neural network and image segmentation, and the specific steps are as follows:
[0070] S1: Structural diagram of scratch recognition and positioning network figure 2 As shown, according to the morphological characteristics of the scratches, a multi-feature fusion module consisting of an upsampling layer, a horizontal connection layer and a feature fusion layer is designed. The horizontal connection layer is fused, and the 6-layer feature layer used for prediction in the SSD network is respectively input into 6 multi-feature fusion modules, and the feature layer output by the multi-feature fusion module is used for prediction in step S2, image 3 It is a multi-feature fusion module structure diagram;
[0071] Specifically, ...
PUM
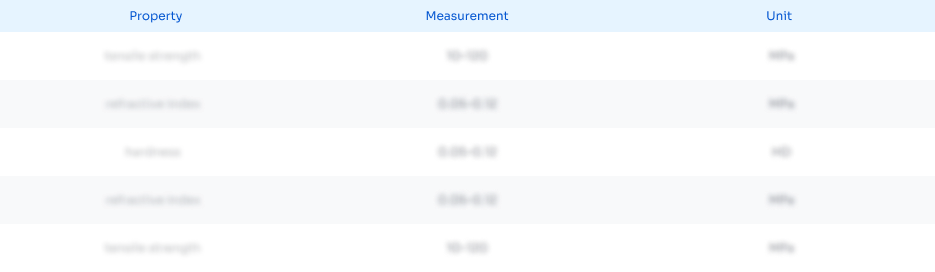
Abstract
Description
Claims
Application Information
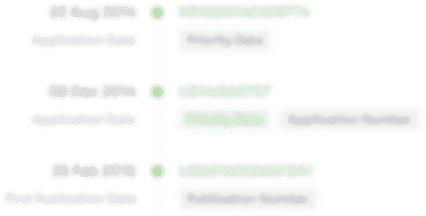
- R&D Engineer
- R&D Manager
- IP Professional
- Industry Leading Data Capabilities
- Powerful AI technology
- Patent DNA Extraction
Browse by: Latest US Patents, China's latest patents, Technical Efficacy Thesaurus, Application Domain, Technology Topic.
© 2024 PatSnap. All rights reserved.Legal|Privacy policy|Modern Slavery Act Transparency Statement|Sitemap