Method for realizing analogue circuit fault diagnosis based on standard deviation and skewness by neural network
A technology for simulating circuit faults and neural networks, which is applied in the field of neural networks for fault diagnosis of analog circuits based on standard deviation and skewness, which can solve the problem of low accuracy of fault location and the inability to distinguish whether the parameters of circuit components are within the tolerance range or have occurred There are no faults, no distinction between hard faults and soft faults of the circuit, etc.
Active Publication Date: 2010-11-24
HUNAN UNIV
View PDF2 Cites 13 Cited by
- Summary
- Abstract
- Description
- Claims
- Application Information
AI Technical Summary
Problems solved by technology
However, it is precisely this unified description of fault characteristics that makes it impossible for these methods to distinguish whether the circuit component parameters are within the tolerance range or a fault has occurred, nor can it dist
Method used
the structure of the environmentally friendly knitted fabric provided by the present invention; figure 2 Flow chart of the yarn wrapping machine for environmentally friendly knitted fabrics and storage devices; image 3 Is the parameter map of the yarn covering machine
View moreImage
Smart Image Click on the blue labels to locate them in the text.
Smart ImageViewing Examples
Examples
Experimental program
Comparison scheme
Effect test
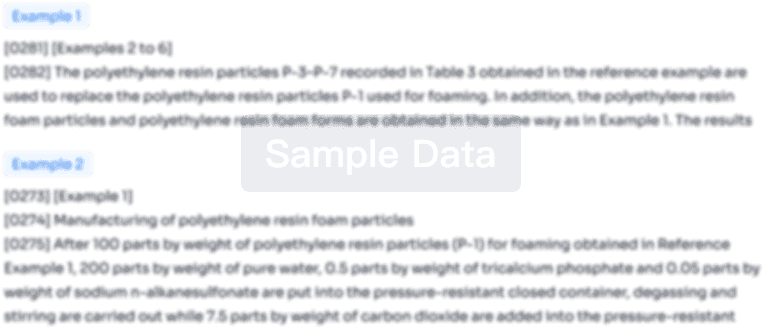
PUM
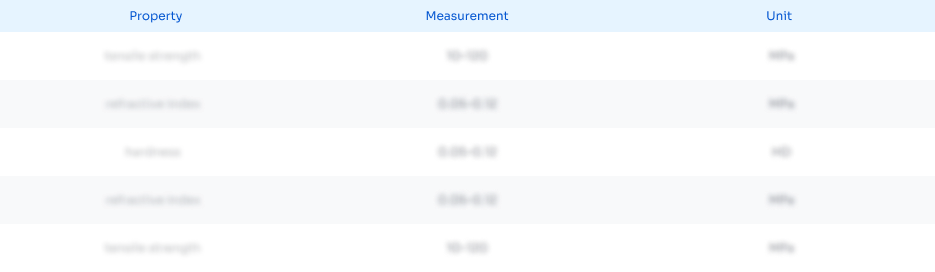
Abstract
The invention discloses a method for realizing analogue circuit fault diagnosis based on standard deviation and skewness by a neural network, which comprises the following steps of: 1) for a circuit under the condition that elements have no tolerance, performing AC analysis in various fault states, extracting amplitude-frequency characteristics and calculating the standard deviation and the skewness to obtain a sample without the tolerance; 2) for the circuit under the condition that the elements have tolerance, performing the AC analysis and Monte Carlo analysis in the various fault states, extracting the amplitude-frequency characteristics and calculating the standard deviation and the skewness to obtain the sample with the tolerance; 3) when a certain element parameter of the circuit is increased from zero to infinity, performing parameter scanning analysis, calculating a track between the standard deviation and the skewness and finding fault characteristic rules; and 4) forming a characteristic vector by the standard deviation and the skewneess obtained in the steps 1) to 3), inputting the characteristic vector into the neural network and performing fault classification. The method has the advantages of avoiding spectrum aliasing, simplifying the structure of the neural network and correctly differentiating the tolerance admissible range state, the soft fault state and the hard fault state of the circuit, along with short network training time and high fault positioning accuracy.
Description
technical field [0001] The invention relates to a neural network method for analog circuit fault diagnosis, in particular to a neural network method for analog circuit fault diagnosis based on standard deviation and skewness. Background technique [0002] Since the start of research on analog circuit fault diagnosis technology in the 1960s, many achievements have been made. Researchers have proposed many methods, among which the artificial neural network is closer to the human brain in terms of composition principles and functional characteristics, and is different from traditional fault diagnosis methods. Compared with the analysis method, it does not need to establish an accurate mathematical model of the object, and avoids complicated mathematical operations, so it has been more and more widely used in fault diagnosis. [0003] The extraction of fault features plays a very important role when neural network is used for fault pattern recognition. The relevant literature o...
Claims
the structure of the environmentally friendly knitted fabric provided by the present invention; figure 2 Flow chart of the yarn wrapping machine for environmentally friendly knitted fabrics and storage devices; image 3 Is the parameter map of the yarn covering machine
Login to view more Application Information
Patent Timeline
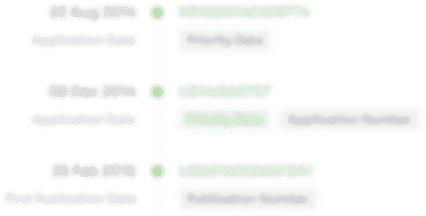
IPC IPC(8): G06N3/02G01R31/316
Inventor 何怡刚祝文姬
Owner HUNAN UNIV
Who we serve
- R&D Engineer
- R&D Manager
- IP Professional
Why Eureka
- Industry Leading Data Capabilities
- Powerful AI technology
- Patent DNA Extraction
Social media
Try Eureka
Browse by: Latest US Patents, China's latest patents, Technical Efficacy Thesaurus, Application Domain, Technology Topic.
© 2024 PatSnap. All rights reserved.Legal|Privacy policy|Modern Slavery Act Transparency Statement|Sitemap