Automatic discovery method of complex system oriented MAXQ task graph structure
A complex system and automatic discovery technology, applied in instruments, electrical digital data processing, computers, etc., can solve the problems that subtasks cannot be further divided, and MAXQ has weak automatic layering ability, etc.
- Summary
- Abstract
- Description
- Claims
- Application Information
AI Technical Summary
Problems solved by technology
Method used
Image
Examples
Embodiment Construction
[0053] The present invention will be described in detail below.
[0054] Assume that the interaction between the Agent and the environment occurs at a series of discrete moments t=0, 1, 2, . . . At each time t, the Agent obtains the state si∈S by observing the environment. Agent chooses the exploration action at ∈ A according to strategy π and executes it. At the next moment t+1, the Agent receives the reinforcement signal (reward value) rt+1∈R from the environment, and reaches a new state st+1∈S. According to the reinforcement signal rt+1, the Agent improves the strategy π. The ultimate goal of reinforcement learning is to find an optimal strategy The state value obtained by the Agent (that is, the total reward obtained by the state) V π (S) Maximum (or minimum), where γ is the remuneration discount factor. 0≤γ≤1. Due to the randomness of the state transition of the environment, under the action of policy π, the state s t value of: where P(s t+1 |s t , a t ) is ...
PUM
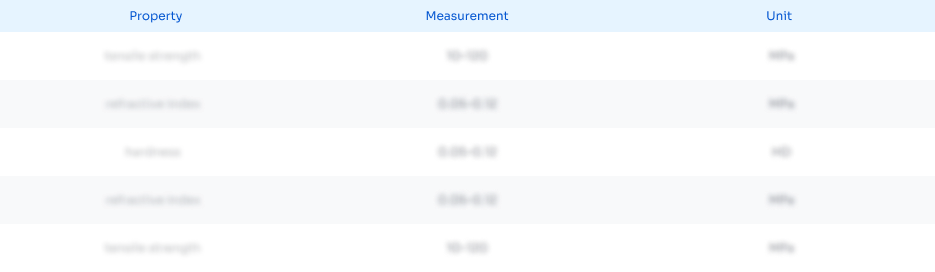
Abstract
Description
Claims
Application Information
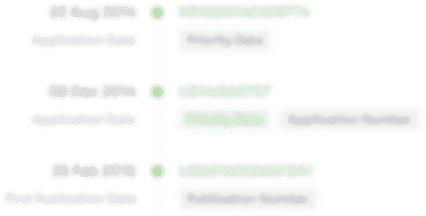
- R&D Engineer
- R&D Manager
- IP Professional
- Industry Leading Data Capabilities
- Powerful AI technology
- Patent DNA Extraction
Browse by: Latest US Patents, China's latest patents, Technical Efficacy Thesaurus, Application Domain, Technology Topic, Popular Technical Reports.
© 2024 PatSnap. All rights reserved.Legal|Privacy policy|Modern Slavery Act Transparency Statement|Sitemap|About US| Contact US: help@patsnap.com