Face recognition method based on dictionary learning models
A dictionary learning and face recognition technology, applied in the field of face recognition based on dictionary learning model, can solve the problems of ignoring the irrelevance degree of the base signal and the difficulty of generalization performance of test data, and achieve the effect of high accuracy
- Summary
- Abstract
- Description
- Claims
- Application Information
AI Technical Summary
Problems solved by technology
Method used
Image
Examples
Embodiment 1
[0036] Step 1: Map the training and testing face images to a low-dimensional space to obtain the training signal set matrix Y.
[0037] The input sample is the face sample picture in the Extended Yale B database, which contains a total of 2414 pictures of 38 people under different lighting conditions. All pictures have been standardized, and the size is 168×192 pixels, such as figure 2shown. Randomly divide each person's sample into two parts, training sample and test sample, and stretch each sample picture into a vector, then normalize it into a unit vector, and then use PCA [4] Reduce all samples to a 504-dimensional space.
[0038] Step 2: Establish a dictionary learning model, input Y into the dictionary learning model, and obtain the dictionary D adapted to the training set, the sparse vector matrix X of the training set, and the linear classifier W. The dictionary learned in this embodiment contains 570 basic signals, and the sparse coefficient threshold T=16.
[003...
Embodiment 2
[0091] This embodiment conducts experiments based on the CAS-PEAL-R1 face database. The CAS-PEAL-R1 face database contains 30,900 images of 1,040 individuals, including pose, expression, occlusion, and illumination changes. Independent experiments were carried out on these four data sets, and 7, 5, 6, 9 training samples and 1 testing sample were selected respectively. The number of sample categories in each experiment is 242, that is, there are 242 different people in the experimental images.
[0092] The operation steps are the same as those in the first embodiment. All samples are first normalized and dimensionally reduced to a 500-dimensional space. The learned dictionary contains 700 basic signals, and the sparse coefficient threshold is set to T=16.
PUM
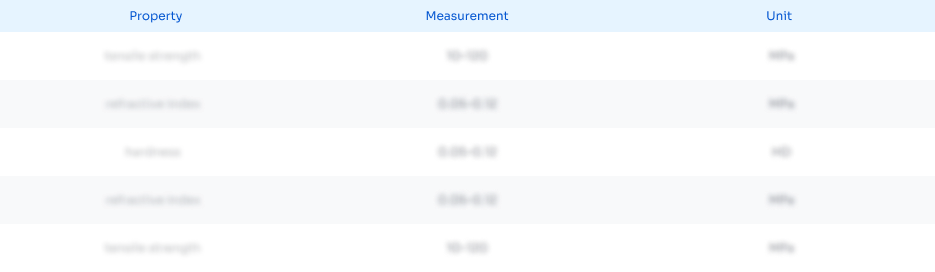
Abstract
Description
Claims
Application Information
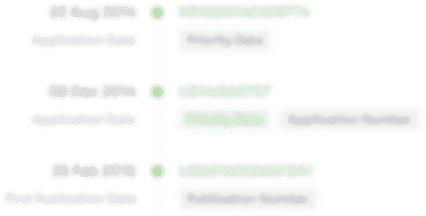
- R&D Engineer
- R&D Manager
- IP Professional
- Industry Leading Data Capabilities
- Powerful AI technology
- Patent DNA Extraction
Browse by: Latest US Patents, China's latest patents, Technical Efficacy Thesaurus, Application Domain, Technology Topic, Popular Technical Reports.
© 2024 PatSnap. All rights reserved.Legal|Privacy policy|Modern Slavery Act Transparency Statement|Sitemap|About US| Contact US: help@patsnap.com