Traffic flow prediction method based on quick learning neural network with double optimal learning rates
A neural network and learning rate technology, which is applied in the field of traffic flow prediction based on double optimal learning rate fast learning neural network, can solve the problems of lagging prediction results and achieve faster convergence, fast network training, and high-precision traffic flow prediction Effect
- Summary
- Abstract
- Description
- Claims
- Application Information
AI Technical Summary
Problems solved by technology
Method used
Image
Examples
Embodiment Construction
[0019] The traffic flow prediction method based on double optimal learning rate fast learning neural network, the specific steps are:
[0020] (1) Input variable selection and preprocessing of the prediction network. In order to predict the traffic flow data at the next moment from the current moment, the nine historical traffic flow data from the current moment to the first are selected as the input of the prediction network. Because the traffic flow changes greatly, the normalization method is adopted, and its range is limited to [-1, 1] through normalization.
[0021] (2) Determination and initialization of the network structure. Theoretically, the three-layer BP network can realize any nonlinear mapping, so a three-layer network structure is adopted, such as figure 1 As shown, J, K, and I represent the input layer, hidden layer, and output layer of the network respectively, and the numbers of neurons are m, L, and 1 respectively, and the hidden layer uses the morlet wave...
PUM
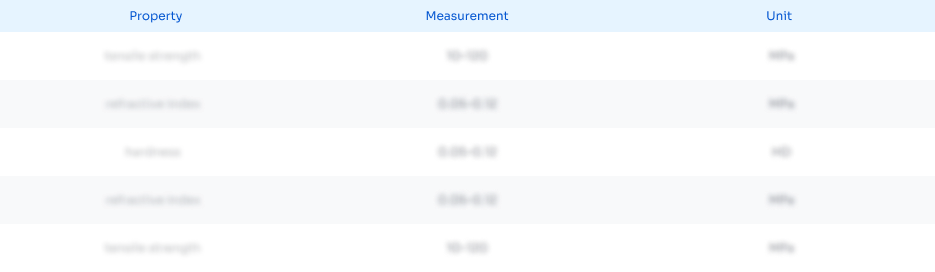
Abstract
Description
Claims
Application Information
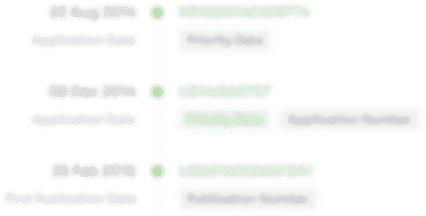
- Generate Ideas
- Intellectual Property
- Life Sciences
- Materials
- Tech Scout
- Unparalleled Data Quality
- Higher Quality Content
- 60% Fewer Hallucinations
Browse by: Latest US Patents, China's latest patents, Technical Efficacy Thesaurus, Application Domain, Technology Topic, Popular Technical Reports.
© 2025 PatSnap. All rights reserved.Legal|Privacy policy|Modern Slavery Act Transparency Statement|Sitemap|About US| Contact US: help@patsnap.com