Deep belief network image recognition method based on Bayesian regularization
A deep learning network and image recognition technology, which is applied in the field of recognition and classification of handwritten digital images, can solve problems that affect the results and are not easy to promote
- Summary
- Abstract
- Description
- Claims
- Application Information
AI Technical Summary
Problems solved by technology
Method used
Image
Examples
Embodiment Construction
[0057] Below in conjunction with specific embodiment, the present invention will be further described;
[0058] see figure 1 Shown is a schematic diagram of the DBN network structure and training principle of the present invention.
[0059] The present invention obtains a DBN network training method based on Bayesian regularization. By introducing Bayesian regularization, the method controls the variation of network weights in the training process, improves the sparseness of weights, and thus improves the network generalization. purpose of capacity.
[0060] The experiment uses the MNIST handwriting database, selects 5,000 samples from the database for training, and selects another 1,000 samples without labels for testing.
[0061] The present invention adopts following technical scheme and implementation steps:
[0062] A deep neural network learning method based on Bayesian regularization, comprising the following steps:
[0063] (1 determine the input object and network...
PUM
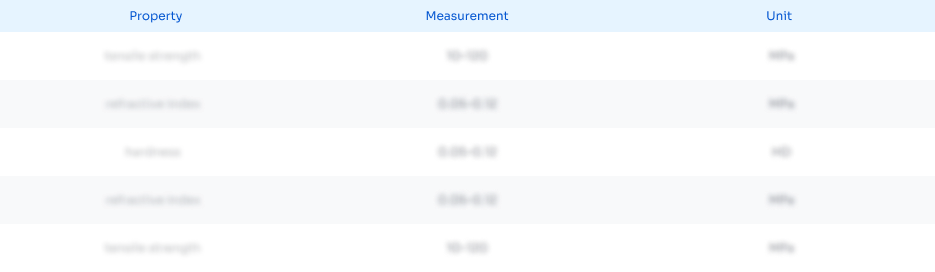
Abstract
Description
Claims
Application Information
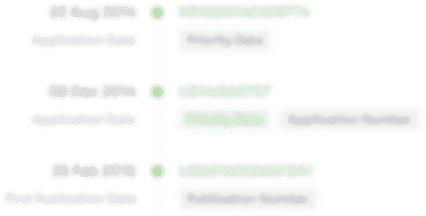
- R&D
- Intellectual Property
- Life Sciences
- Materials
- Tech Scout
- Unparalleled Data Quality
- Higher Quality Content
- 60% Fewer Hallucinations
Browse by: Latest US Patents, China's latest patents, Technical Efficacy Thesaurus, Application Domain, Technology Topic, Popular Technical Reports.
© 2025 PatSnap. All rights reserved.Legal|Privacy policy|Modern Slavery Act Transparency Statement|Sitemap|About US| Contact US: help@patsnap.com