Extreme learning machine-based hyperspectral remote sensing image ground object classification method
An extreme learning machine and hyperspectral remote sensing technology, which is applied in the field of object classification based on hyperspectral remote sensing images, can solve the problems of insufficient accuracy of object classification, reduce the risk of over-fitting, shorten the training time, and improve the training speed. fast effect
- Summary
- Abstract
- Description
- Claims
- Application Information
AI Technical Summary
Problems solved by technology
Method used
Image
Examples
Embodiment Construction
[0041] The present invention will be described in detail below with reference to the accompanying drawings and examples. The method model involved in the present invention can be provided by the attached figure 1 shown.
[0042] Step 1, collect hyperspectral image data samples, and form a training set after preprocessing; wherein, the training set includes the spectral information, spatial information and category identification of the target;
[0043] Spectral information preprocessing mainly refers to scale-normalized spectral information data. Firstly, the spectral vector of the pixel corresponding to the object in the hyperspectral image is extracted, and the dimension of the vector is equal to the number of image spectral segments. The multiple spectral vectors of ground objects form a spectral array, and the value of each dimension is scale-normalized so that the mean value is 0 and the variance is 1 (the zscore function in Matlab can accurately realize this process). ...
PUM
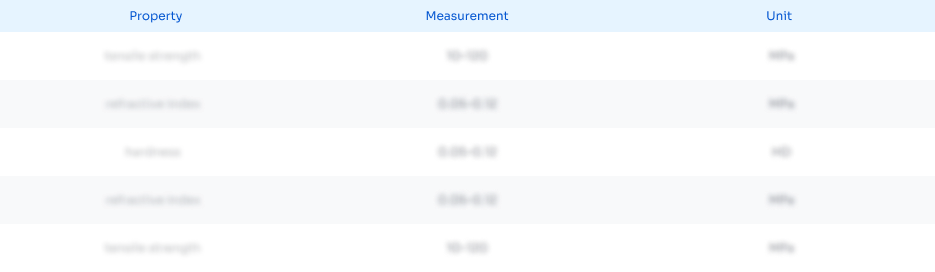
Abstract
Description
Claims
Application Information
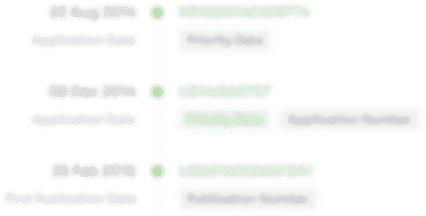
- R&D
- Intellectual Property
- Life Sciences
- Materials
- Tech Scout
- Unparalleled Data Quality
- Higher Quality Content
- 60% Fewer Hallucinations
Browse by: Latest US Patents, China's latest patents, Technical Efficacy Thesaurus, Application Domain, Technology Topic, Popular Technical Reports.
© 2025 PatSnap. All rights reserved.Legal|Privacy policy|Modern Slavery Act Transparency Statement|Sitemap|About US| Contact US: help@patsnap.com