Classification of cancers
A cancer, brain cancer technology, applied in the field of cancer classification, can solve problems such as inability to represent molecular subtypes, limiting individual cancers, etc.
- Summary
- Abstract
- Description
- Claims
- Application Information
AI Technical Summary
Problems solved by technology
Method used
Image
Examples
Embodiment 1
[0092] Meta-analysis of gene expression in cancer
[0093] Analysis of gene expression in cancer patients was obtained from the Oncomine database at http: / / www.oncomine.org, and processed and normalized as described in Rhodes et al., Neoplasia, 2007 Feb;9(2):166-80. Data sets from the 15 most representative cancer types were analyzed. Average linkage hierarchical clustering using the Poisson correlation coefficient as a distance measure was performed on each data set. Up to 10,000 features with the largest standard deviation (but not more than 50% of the total features) were included in the analysis. To reduce the hierarchical clustering results of discrete gene expression clusters, clusters with the most features were identified with a minimum Poisson correlation coefficient of 0.5 and a minimum of 10 features (Rhodes, Neoplasia, 2007). Based on Fisher's exact test, pairwise correlation analysis was performed for each pair of clusters, the number of overlapping genes was co...
Embodiment 2
[0095] Identification of Cancer Modules
[0096] Paired cluster correlations were visualized using a network representation (Cytoscape) and modules of highly connected clusters were identified. To reduce the cluster correlation network into discrete modular groups, the edges without at least two supporting indirect associations are removed, and the nodes and edges connecting the two groups of connected clusters that are least connected if not removed are removed. Define each cancer module as a list of contiguous clusters.
[0097] For each module, representative genes are ranked based on the number of clusters of which they are members. The cancer modules identified for the 15 cancer types are shown in Tables 1-161.
Embodiment 3
[0099] Identification of cancers belonging to the cancer module using quantitative RT-PCR
[0100] Tumor biopsies are obtained from patients. use Oligo(dT) 25 mRNA Purification Kit (Invitrogen, Carlsbad, CA) purifies messenger RNA from the biopsies according to the manufacturer's instructions. Briefly, tumor cells were lysed by grinding the samples in liquid nitrogen to form crude lysates. The lysate was added to the washed Oligo(dT) 25 The beads were incubated at room temperature to allow the poly-A mRNA to anneal to the beads. The beads with bound mRNA are recovered using a magnet, washing away other cellular components. Then, the mRNA was eluted from the beads and used in RT-PCR.
[0101] Use RETRO according to the manufacturer's two-step RT-PCR protocol cDNA kit ( Austin, TX) reverse transcribed the purified mRNA. Briefly, 20-200 ng of mRNA was mixed with 10 base random primers and denatured at 85°C. The primers were then annealed to the mRNA template on ice...
PUM
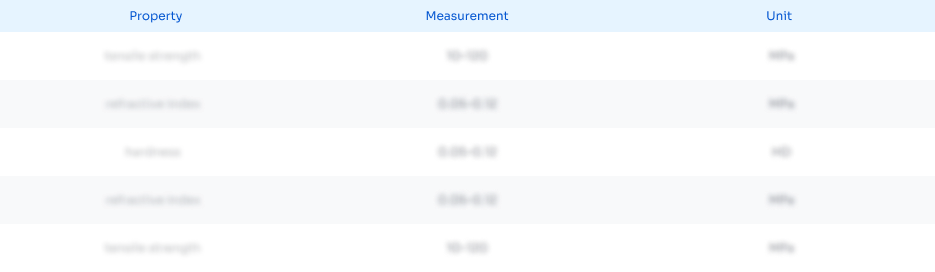
Abstract
Description
Claims
Application Information
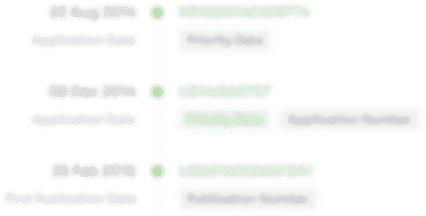
- R&D Engineer
- R&D Manager
- IP Professional
- Industry Leading Data Capabilities
- Powerful AI technology
- Patent DNA Extraction
Browse by: Latest US Patents, China's latest patents, Technical Efficacy Thesaurus, Application Domain, Technology Topic, Popular Technical Reports.
© 2024 PatSnap. All rights reserved.Legal|Privacy policy|Modern Slavery Act Transparency Statement|Sitemap|About US| Contact US: help@patsnap.com