Classifier construction method based on active learning
A technology of active learning and construction methods, applied in the field of data classification, can solve the problems that the training results cannot meet the expected goals, and achieve the effect of low time complexity, high classification performance, and optimized calculation methods
- Summary
- Abstract
- Description
- Claims
- Application Information
AI Technical Summary
Problems solved by technology
Method used
Image
Examples
Embodiment 1
[0039] Example 1: see figure 1 As shown, a method for constructing a classifier based on active learning, generating a training sample set and training a classifier based on unlabeled samples and data features, includes the following steps:
[0040] (1) Randomly select 20-50 samples from the unlabeled sample set for manual labeling, construct the initial training sample set, and then build the initial classifier H according to the data characteristics of the initial training sample set (0) ;
[0041] (2) Using the classifier H obtained in the previous step (t) Calculate the BvSB value of each unlabeled sample. The calculation method of the BvSB value is:
[0042] ,
[0043] Where x is the sample in the unlabeled sample set U, Is the posterior probability that the sample belongs to the optimal category, Is the posterior probability that the sample belongs to the sub-optimal category, and t is the number of cycles from steps (2) to (6);
[0044] (3) According to step (2), select h unla...
PUM
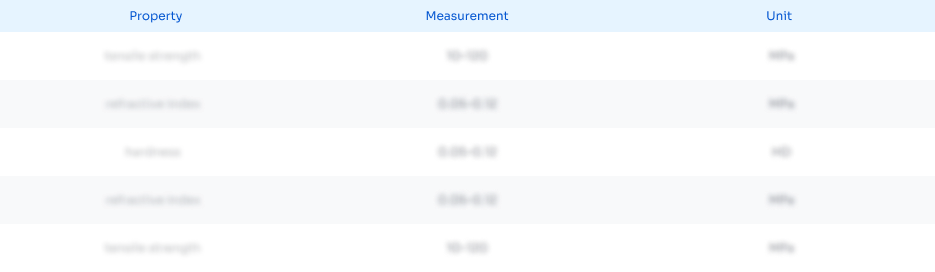
Abstract
Description
Claims
Application Information
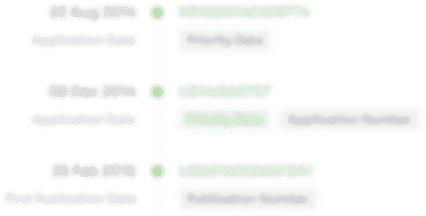
- R&D Engineer
- R&D Manager
- IP Professional
- Industry Leading Data Capabilities
- Powerful AI technology
- Patent DNA Extraction
Browse by: Latest US Patents, China's latest patents, Technical Efficacy Thesaurus, Application Domain, Technology Topic, Popular Technical Reports.
© 2024 PatSnap. All rights reserved.Legal|Privacy policy|Modern Slavery Act Transparency Statement|Sitemap|About US| Contact US: help@patsnap.com