Automatic classification method for electrocardiogram signals
A technology for automatic classification of ECG signals, applied in the direction of diagnostic signal processing, medical science, sensors, etc., can solve problems such as unstable classification of ECG signals, and achieve the problem of unstable classification algorithms, good stability, stable and accurate identification Effect
- Summary
- Abstract
- Description
- Claims
- Application Information
AI Technical Summary
Benefits of technology
Problems solved by technology
Method used
Image
Examples
Embodiment 1
[0047] This embodiment is implemented in a computer with Intel Xeon CPU E5-2697 2.70GHz, memory 128.00GB, Win7, 64-bit operating system, and the entire ECG signal automatic classification algorithm is implemented in Matlab language.
[0048] The implementation process of the present invention is as figure 1 Shown:
[0049] a) Obtain the original ECG signal of the human body, perform filtering processing, and detect the R wave of the filtered ECG signal, which specifically operates according to the following steps:
[0050] (1) ECG original signal collection: The present invention utilizes the MedSun 18-lead Holter of Beijing Pengyang Fengye to collect the ECG signal of the human body for a long time, and its sampling output frequency is 250 Hz, and the collected ECG data is stored in the form of TXT. It can be easily read into the Matlab environment for display, and its form is as follows figure 2 .
[0051] (2) Filtering the collected ECG raw signal data:
[0052] (2-1) De...
PUM
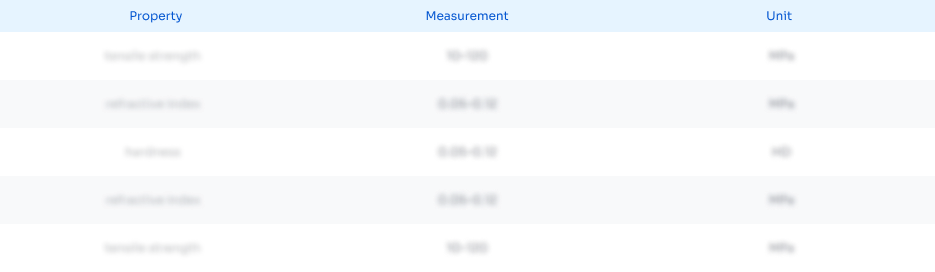
Abstract
Description
Claims
Application Information
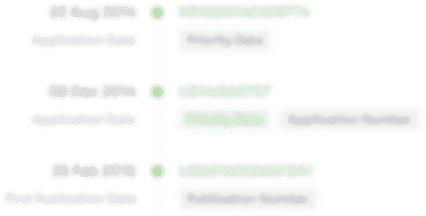
- Generate Ideas
- Intellectual Property
- Life Sciences
- Materials
- Tech Scout
- Unparalleled Data Quality
- Higher Quality Content
- 60% Fewer Hallucinations
Browse by: Latest US Patents, China's latest patents, Technical Efficacy Thesaurus, Application Domain, Technology Topic, Popular Technical Reports.
© 2025 PatSnap. All rights reserved.Legal|Privacy policy|Modern Slavery Act Transparency Statement|Sitemap|About US| Contact US: help@patsnap.com