Method for transforming input signal
A technology of input signals and variables, applied in the field of signal processing, can solve problems such as unrealistic independence assumptions
- Summary
- Abstract
- Description
- Claims
- Application Information
AI Technical Summary
Problems solved by technology
Method used
Image
Examples
Embodiment Construction
[0063] introduction
[0064] Our embodiments provide models for transforming and processing dynamic (non-stationary) signals and data that have the advantages of HMM and NMF based models.
[0065] The model is characterized by a continuous non-negative state space. Gain adaptation is automatically handled in real-time during inference. The dynamics of the signal were modeled using a linear transfer matrix A. The model is a random variable ε with multiplicative non-negative innovation n non-negative linear dynamical system. The signal may be a non-stationary linear signal (such as an audio or speech signal) or a multidimensional signal. The signal may be represented as data in the digital domain. The innovation random variable is described in more detail below.
[0066] The embodiments also provide applications for using the models. In particular, the model can be used to process audio signals taken from several sources, e.g. the signal is a mixture of speech and noise (...
PUM
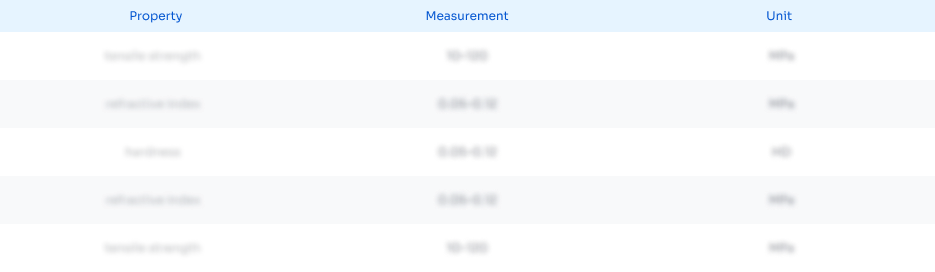
Abstract
Description
Claims
Application Information
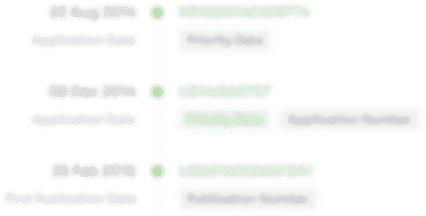
- R&D
- Intellectual Property
- Life Sciences
- Materials
- Tech Scout
- Unparalleled Data Quality
- Higher Quality Content
- 60% Fewer Hallucinations
Browse by: Latest US Patents, China's latest patents, Technical Efficacy Thesaurus, Application Domain, Technology Topic, Popular Technical Reports.
© 2025 PatSnap. All rights reserved.Legal|Privacy policy|Modern Slavery Act Transparency Statement|Sitemap|About US| Contact US: help@patsnap.com