Dense crowd estimation method based on deep learning
A deep learning and headcount technology, applied in the field of intensive headcount estimation based on deep learning, can solve problems such as difficult to apply, hard to detect, time-consuming, etc., and achieve the effect of reducing the possibility of overfitting
- Summary
- Abstract
- Description
- Claims
- Application Information
AI Technical Summary
Problems solved by technology
Method used
Image
Examples
Embodiment Construction
[0030] The present invention will now be further described in conjunction with specific examples, and the following examples are intended to illustrate the present invention rather than further limit the present invention.
[0031] 1. Data collection
[0032] The data comes from the Google image search engine, from which 107 images of dense scenes are selected as a data set. The number of people contained in the data set ranges from 58-2201, and then the crowds in the images are manually marked (each person is represented by a point ), and finally we slice the image, and normalize each block to a small pixel block of 32×32, attach the corresponding label, the label contains the specific number of people in the image block and the corresponding density level (the density level is based on The number of people in the image block to delineate), such as figure 2 shown.
[0033] Then, the method of horizontal mirroring and horizontal and vertical offset is used to enhance the da...
PUM
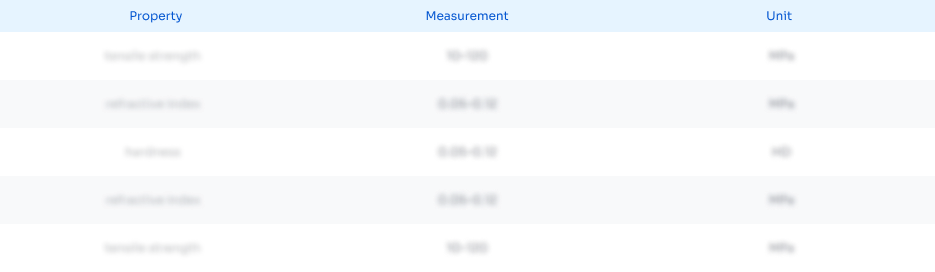
Abstract
Description
Claims
Application Information
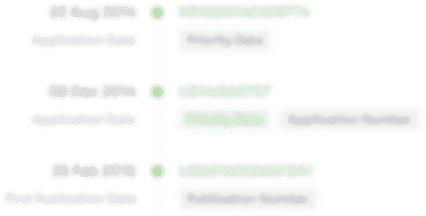
- R&D
- Intellectual Property
- Life Sciences
- Materials
- Tech Scout
- Unparalleled Data Quality
- Higher Quality Content
- 60% Fewer Hallucinations
Browse by: Latest US Patents, China's latest patents, Technical Efficacy Thesaurus, Application Domain, Technology Topic, Popular Technical Reports.
© 2025 PatSnap. All rights reserved.Legal|Privacy policy|Modern Slavery Act Transparency Statement|Sitemap|About US| Contact US: help@patsnap.com