Data flow detection method based on fuzzy C-means clustering algorithm and entropy theory
A mean value clustering and detection method technology, which is applied in computing, computer parts, character and pattern recognition, etc., can solve problems such as performance degradation and data that cannot be processed as accurately as possible
- Summary
- Abstract
- Description
- Claims
- Application Information
AI Technical Summary
Problems solved by technology
Method used
Image
Examples
Embodiment 1
[0032] We selected an artificial data set and two real data for experiments. The real data is downloaded from the open database UCI. The first is real data without concept drift, SeedsData data set, this data set includes three categories, namely Kama, Rosa and Canadian, each category has 70 samples and seven attributes. From figure 1 It can be seen that FCM clustered the data more accurately; figure 2 It is the entropy curve of the data. It can be seen from the ordinate that when the concept drift occurs with good classification and no attribute changes, the entropy value is relatively low.
Embodiment 2
[0034] The Gaussian data set is used to detect concept drift. The two sets of Gaussian data obey the distribution of N([2;2],1) and N([4;4],8). The data stream length is 1000, and the conceptual drift length is 400. image 3 It is the classification of the two sets of Gaussian data. Because the mean and variance are different, it shows that the attributes of the data have changed, and the concept drift of attribute changes has occurred in the junction part. Figure 4 Is the curve of its data flow entropy. It can be seen that the peak of the entropy curve appears at the junction, indicating that the conceptual drift of the attribute change has occurred; after that, the entropy value tends to be stable again, indicating that the current system can adapt to the new data stream and does not require parameter updates.
Embodiment 3
[0036] Powersupply data set. This data set collects 24-hour power supply data for the main network and subnet. There are 1247 samples per hour. The experiment selected data for three time periods: 0 o'clock, 1 o'clock, and 21 o'clock. The first is to experiment with the data of 0 o'clock and 21 o'clock. Compared with 0 o'clock, 21 o'clock is the peak point of electricity consumption. It can be considered that the concept drift of attribute change has occurred compared with 0 o'clock. Figure 5 It is the entropy curve of the two sets of data at the junction. It can be seen that the entropy value increases significantly. After the data is stable, the entropy value decreases. Image 6 It is the data flow entropy curve at 0 and 1 point. The electricity consumption at 0 and 1 point is similar. It can be regarded as a data flow without conceptual drift, so the entropy curve is stable.
PUM
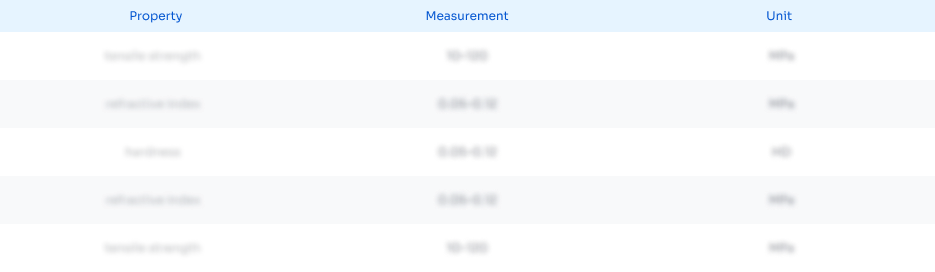
Abstract
Description
Claims
Application Information
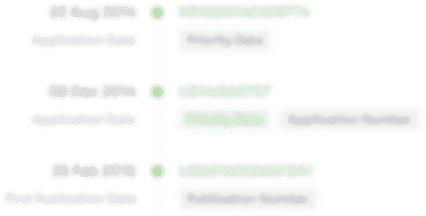
- R&D Engineer
- R&D Manager
- IP Professional
- Industry Leading Data Capabilities
- Powerful AI technology
- Patent DNA Extraction
Browse by: Latest US Patents, China's latest patents, Technical Efficacy Thesaurus, Application Domain, Technology Topic.
© 2024 PatSnap. All rights reserved.Legal|Privacy policy|Modern Slavery Act Transparency Statement|Sitemap