Image segmentation method based on hierarchical higher order conditional random field
A conditional random field and image segmentation technology, applied in image analysis, image enhancement, image data processing, etc., can solve the problems of segmentation result dependence, inappropriate segmentation granularity, random field model of segmentation accuracy, etc.
- Summary
- Abstract
- Description
- Claims
- Application Information
AI Technical Summary
Problems solved by technology
Method used
Image
Examples
Embodiment Construction
[0028] The present invention will be further described in detail below in conjunction with the accompanying drawings and specific embodiments.
[0029] 1. Extraction of image pixel-level features
[0030] (1) Texture features
[0031] The present invention adopts the method based on the filter group proposed by Malik et al. First, the image is converted from the RGB color space to the CIE-Lab color space, and then a 17-dimensional multi-channel multi-scale Gaussian filter group is used to extract each pixel point The texture information, the filter set includes the basic Gaussian model filter under different scales and channels, the first-order partial derivative filter in the X and Y directions, and the Laplacian filter, then each pixel is associated with a 17 dimensional feature vector, each vector contains the area texture information of the corresponding pixel. Finally, a pixel is associated with a 17-dimensional vector, which is used as the texture feature of the image....
PUM
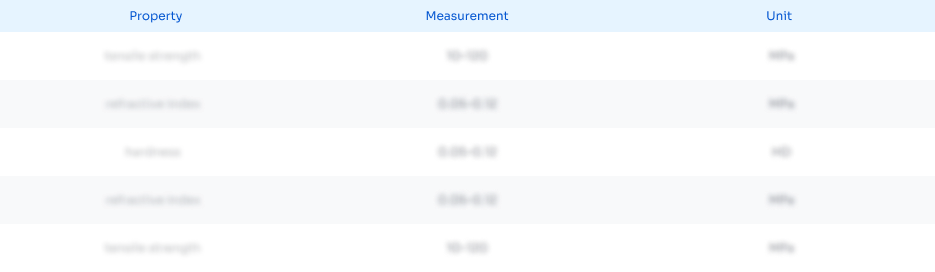
Abstract
Description
Claims
Application Information
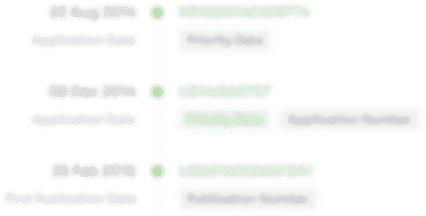
- R&D Engineer
- R&D Manager
- IP Professional
- Industry Leading Data Capabilities
- Powerful AI technology
- Patent DNA Extraction
Browse by: Latest US Patents, China's latest patents, Technical Efficacy Thesaurus, Application Domain, Technology Topic, Popular Technical Reports.
© 2024 PatSnap. All rights reserved.Legal|Privacy policy|Modern Slavery Act Transparency Statement|Sitemap|About US| Contact US: help@patsnap.com