Kernel-based possibilistic c-means clustering method of maximum central interval
A mean-value clustering and probability technique, applied in the fields of instruments, character and pattern recognition, computer parts, etc., can solve the problems of ignoring the distance relationship between class centers, data misclassification, and overlapping or offset of cluster center positions.
- Summary
- Abstract
- Description
- Claims
- Application Information
AI Technical Summary
Problems solved by technology
Method used
Image
Examples
Embodiment Construction
[0031] In order to demonstrate the purpose and advantages of the present invention more clearly and easily, the present invention will be further described in detail below in conjunction with the accompanying drawings and specific embodiments.
[0032] The present invention provides a nuclear possibility C-means clustering method with a maximum center interval. On the basis of the KPCM algorithm, the present invention introduces a maximum penalty term and a regulation factor λ between classes in a high-dimensional space to construct a brand-new objective function , by adjusting the regulatory factors to control the distance between the clusters, avoiding the coincidence or even offset of the cluster centers. During the experiment, the clustering analysis is carried out according to the distance relationship of the sample points and the pixel value of the pixel points, and it has a better clustering effect in dealing with the sample points with blurred boundaries and closer gray...
PUM
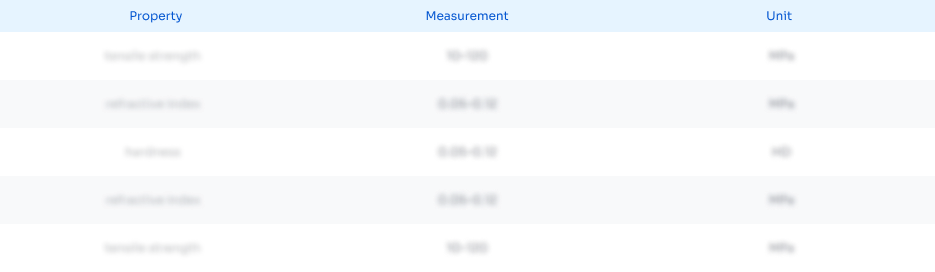
Abstract
Description
Claims
Application Information
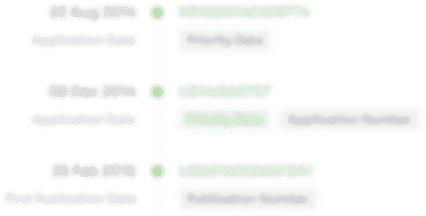
- R&D
- Intellectual Property
- Life Sciences
- Materials
- Tech Scout
- Unparalleled Data Quality
- Higher Quality Content
- 60% Fewer Hallucinations
Browse by: Latest US Patents, China's latest patents, Technical Efficacy Thesaurus, Application Domain, Technology Topic, Popular Technical Reports.
© 2025 PatSnap. All rights reserved.Legal|Privacy policy|Modern Slavery Act Transparency Statement|Sitemap|About US| Contact US: help@patsnap.com