Image segmentation method converting unsupervised cluster into self-supervised classification
A technology for supervised classification and image segmentation, which is applied in image analysis, image data processing, instrumentation, etc., and can solve problems such as unacceptable segmentation results.
- Summary
- Abstract
- Description
- Claims
- Application Information
AI Technical Summary
Problems solved by technology
Method used
Image
Examples
Embodiment Construction
[0024] In order to make the content of the present invention clearer and easier to understand, the content of the present invention will be described in detail below in conjunction with specific embodiments and accompanying drawings.
[0025] The existing method is to cluster the features without any training samples, that is, to use the so-called unsupervised learning method. Since there is no guidance from the training samples, the robustness of the segmentation results is significantly lower than that obtained by using a classifier trained on typical samples. Therefore, we can try to find some typical training samples to guide the segmentation, and change the original unsupervised learning method into a self-supervised learning method, thereby improving the robustness of the algorithm.
[0026] Moreover, in the segmentation process, it is usually assumed that all sample features belong to the same Gaussian distribution, and there is no division of subspaces. When the real ...
PUM
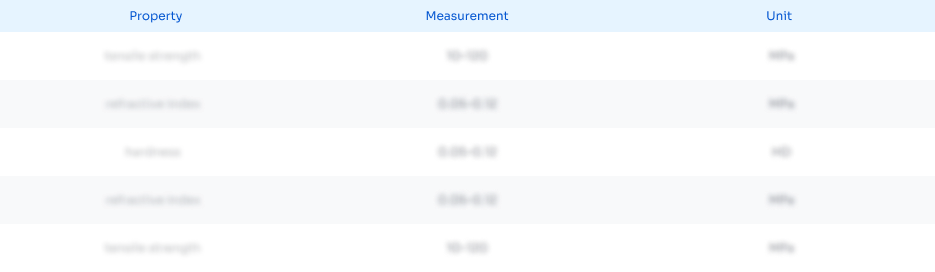
Abstract
Description
Claims
Application Information
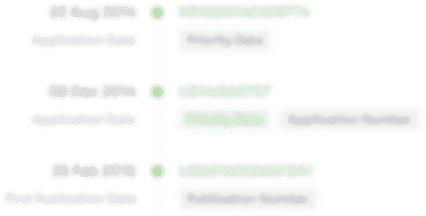
- R&D Engineer
- R&D Manager
- IP Professional
- Industry Leading Data Capabilities
- Powerful AI technology
- Patent DNA Extraction
Browse by: Latest US Patents, China's latest patents, Technical Efficacy Thesaurus, Application Domain, Technology Topic, Popular Technical Reports.
© 2024 PatSnap. All rights reserved.Legal|Privacy policy|Modern Slavery Act Transparency Statement|Sitemap|About US| Contact US: help@patsnap.com