A Semi-supervised Multi-View Clustering Method Based on Structural Constraints
A technology of structural constraints and clustering methods, applied in the field of pattern recognition, can solve problems such as inability to guide multi-view clustering, fail to effectively improve clustering performance, and cannot effectively satisfy semi-supervised multi-view clustering, etc., to achieve effective clustering class effect
- Summary
- Abstract
- Description
- Claims
- Application Information
AI Technical Summary
Problems solved by technology
Method used
Image
Examples
Embodiment Construction
[0016] In order to make the object, technical solution and advantages of the present invention clearer, the present invention will be described in further detail below in conjunction with specific embodiments and with reference to the accompanying drawings.
[0017] see figure 1 As shown, a semi-supervised multi-view clustering method based on structural constraints, including steps:
[0018] Step S1, collecting multi-view data and extracting features of different view data;
[0019] Step S2, manually labeling the similarity relationship between some samples as prior information;
[0020] Step S3, concatenate the features of the multi-view data, and use the linear projection matrix to learn the normalized category matrix of the multi-view data in a regression manner;
[0021] Step S4, designing a regularization method of the linear projection matrix to learn feature weights from different perspectives, and constructing an optimization goal;
[0022] Step S5, adding prior in...
PUM
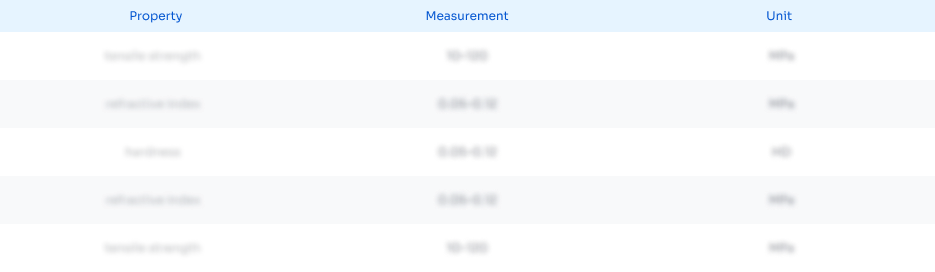
Abstract
Description
Claims
Application Information
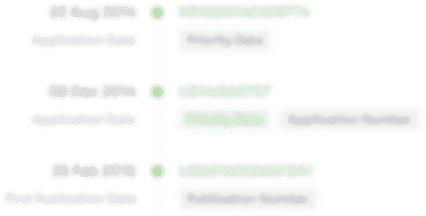
- R&D Engineer
- R&D Manager
- IP Professional
- Industry Leading Data Capabilities
- Powerful AI technology
- Patent DNA Extraction
Browse by: Latest US Patents, China's latest patents, Technical Efficacy Thesaurus, Application Domain, Technology Topic.
© 2024 PatSnap. All rights reserved.Legal|Privacy policy|Modern Slavery Act Transparency Statement|Sitemap