Tensor hyperspectral image spectrum-space dimensionality reduction method based on deep convolutional neural network
A technology of hyperspectral image and deep convolution, applied in image data processing, graphics and image conversion, instruments, etc., can solve the problems of destroying the image structure, not being able to fully utilize the DCNN feature extraction ability, and the disaster of dimensionality
- Summary
- Abstract
- Description
- Claims
- Application Information
AI Technical Summary
Problems solved by technology
Method used
Image
Examples
Embodiment Construction
[0058] The present invention will be further described below in conjunction with the accompanying drawings.
[0059] like figure 1 As shown, a tensor-type hyperspectral image spectral-space dimensionality reduction method based on deep convolutional neural network, first of all, in view of the direct use of high-band tensor data will greatly increase the parameter space of deep convolutional neural network, Introduce the maximum likelihood eigendimension estimation algorithm and principal component analysis to reduce the dimensionality of the hyperspectral image; then, convert the hyperspectral image into a tensor form through the window field, and keep the spectral and spatial information of each pixel; finally , using a deep convolutional neural network to perform spectral-spatial dimensionality reduction on tensor-type hyperspectral images, so that the features after dimensionality reduction include both spectral information and spatial information. Specific steps are as f...
PUM
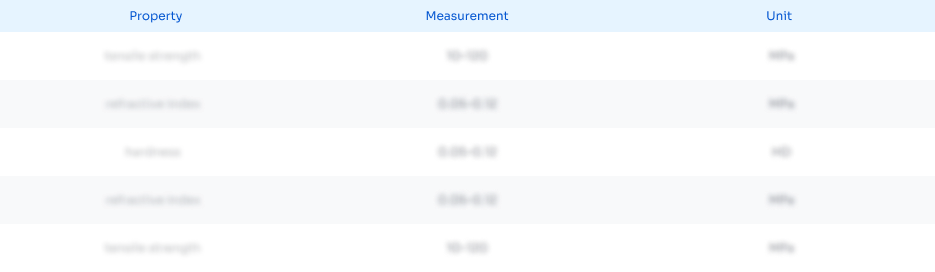
Abstract
Description
Claims
Application Information
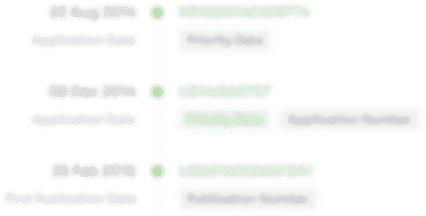
- R&D Engineer
- R&D Manager
- IP Professional
- Industry Leading Data Capabilities
- Powerful AI technology
- Patent DNA Extraction
Browse by: Latest US Patents, China's latest patents, Technical Efficacy Thesaurus, Application Domain, Technology Topic, Popular Technical Reports.
© 2024 PatSnap. All rights reserved.Legal|Privacy policy|Modern Slavery Act Transparency Statement|Sitemap|About US| Contact US: help@patsnap.com