A Nonlocal Mean Denoising Optimization Method Based on Structural Similarity
A non-local mean and structural similarity technology, which is applied in image enhancement, image analysis, instruments, etc., can solve the problems of large difference in denoising performance with different noise intensities, poor image detail processing ability, strong noise denoising ability decline, etc.
- Summary
- Abstract
- Description
- Claims
- Application Information
AI Technical Summary
Problems solved by technology
Method used
Image
Examples
Embodiment 1
[0064] A non-local mean denoising optimization method based on structural similarity. The specific steps of the method are:
[0065] Step 1. Select an image such as figure 1 The noise-contaminated image X shown, figure 1 It is a noise-contaminated image to be denoised in this embodiment. The size of the noise-contaminated image X is 256×256, and the noise standard deviation is 25. Let any pixel i,j∈X,(m,n) be the coordinates of any pixel, select a pixel i in the noise-polluted image X, and take the pixel i as the center to establish a 7×7 noise image search box.
[0066] Step 2. Take a 3×3 noise image similarity box X in the noise image search box t , with the noise image similarity box X t Swipe in the noise image search box to find all noise image similar boxes X in the noise image search box t A combination of similar boxes X recorded for each noisy image t The pixel point j in the center is in the noise image search box centered on the pixel point i, that is, the w...
Embodiment 2
[0119] A non-local mean denoising optimization method based on structural similarity. The specific steps of the method are:
[0120] Step 1. Select an image such as image 3 The noise-contaminated image X shown, image 3 It is a noise-contaminated image to be denoised in this embodiment. The size of the noise-contaminated image X is 256×256, and the noise standard deviation is 25. Let any pixel i,j∈X,(m,n) be the coordinates of any pixel, select a pixel i in the noise-polluted image X, and take the pixel i as the center to establish a 7×7 noise image search box.
[0121] Step 2. Take a 3×3 noise image similarity box X in the noise image search box t , with the noise image similarity box X t Swipe in the noise image search box to find all noise image similar boxes X in the noise image search box t A combination of similar boxes X recorded for each noisy image t The pixel point j in the center is in the noise image search box centered on the pixel point i, that is, the w...
PUM
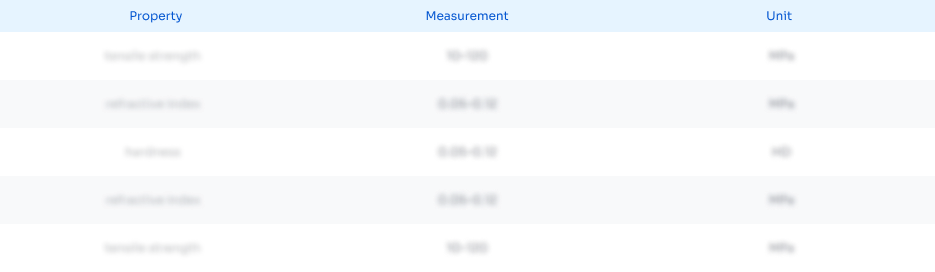
Abstract
Description
Claims
Application Information
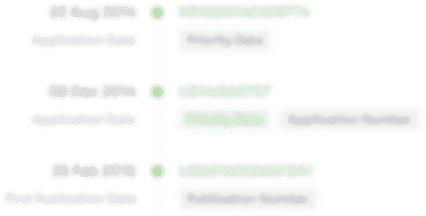
- R&D Engineer
- R&D Manager
- IP Professional
- Industry Leading Data Capabilities
- Powerful AI technology
- Patent DNA Extraction
Browse by: Latest US Patents, China's latest patents, Technical Efficacy Thesaurus, Application Domain, Technology Topic, Popular Technical Reports.
© 2024 PatSnap. All rights reserved.Legal|Privacy policy|Modern Slavery Act Transparency Statement|Sitemap|About US| Contact US: help@patsnap.com