On-line battery soh estimation method based on fractional order neural network and dual volume Kalman
A neural network and neural network model technology, applied in the field of battery health management, can solve the problems of slow training speed and inability to converge, and achieve the effects of fast convergence speed, effective function approximation ability, and high prediction accuracy
- Summary
- Abstract
- Description
- Claims
- Application Information
AI Technical Summary
Problems solved by technology
Method used
Image
Examples
Embodiment Construction
[0050] The invention will be described in further detail below in conjunction with the accompanying drawings.
[0051] The schematic diagram of online estimation of battery dynamic system is as follows: figure 1 Shown, concrete operation of the present invention comprises the following steps:
[0052] In step 1, the visible state of the dynamic system is measured by sensors.
[0053] In step 2, the historical data of the same type of battery is used as the initial training data set, and the neural network algorithm improved by the fractional order theory is used to train the model, and an equivalent model representing the nonlinear relationship of the battery system is obtained.
[0054] The flowchart of the fractional order neural network algorithm in step 2 is as follows figure 2 As shown, the specific process is as follows:
[0055] Step 2.1, the topology of the fractional order neural network is a three-layer network structure (1 input layer, 1 hidden layer and 1 outpu...
PUM
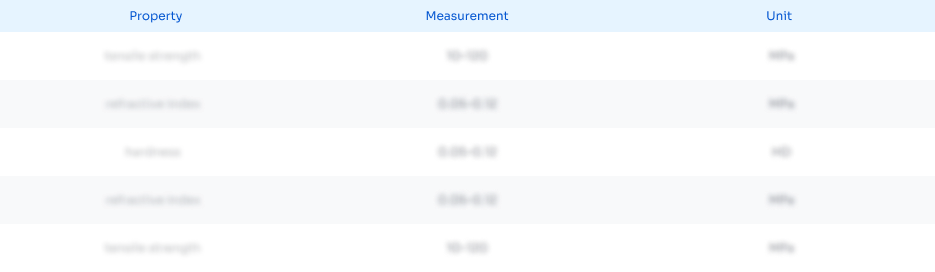
Abstract
Description
Claims
Application Information
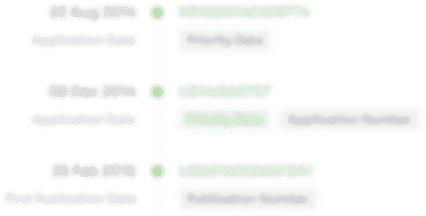
- R&D Engineer
- R&D Manager
- IP Professional
- Industry Leading Data Capabilities
- Powerful AI technology
- Patent DNA Extraction
Browse by: Latest US Patents, China's latest patents, Technical Efficacy Thesaurus, Application Domain, Technology Topic, Popular Technical Reports.
© 2024 PatSnap. All rights reserved.Legal|Privacy policy|Modern Slavery Act Transparency Statement|Sitemap|About US| Contact US: help@patsnap.com