Cascading-type composition generating method
A cascading, compositional technology, applied in unstructured text data retrieval, text database clustering/classification, instruments, etc.
- Summary
- Abstract
- Description
- Claims
- Application Information
AI Technical Summary
Problems solved by technology
Method used
Image
Examples
specific Embodiment approach 1
[0030] Specific implementation mode 1: This implementation mode provides a method for generating a cascading composition, including:
[0031] Step 1. According to the input material composition question stem, extract the topic word set.
[0032] For a given composition topic (topic), we first need to clarify what kind of composition the topic (topic) requires us to write. Through the analysis of the stems of the composition of the college entrance examination, the stems of the questions can be divided into the following three categories:
[0033] ① Topic composition. In the question stem, it is clearly required what topic the essay should meet. For example, please write an essay on the topic of "voice".
[0034] ②Propositional and semi-propositional composition. The title of the composition is clearly required in the question stem (propositional composition), or a part of the topic is given, and candidates need to incomplete the topic (semi-propositional composition). For ...
specific Embodiment approach 2
[0060] Specific implementation mode two: the difference between this implementation mode and specific implementation mode one is that step one specifically includes:
[0061] Step one one, the word vector W in each sentence in the first training corpus i Input to the first GRU model to get the sentence vector representation of each sentence
[0062] Step 12. Represent the sentence vector of each sentence Input to the second GRU model to obtain the vector representation V of the entire text doc ;
[0063] Step 13. Express the vector of the entire text as V doc And the pre-built vocabulary is input to the decoder, and the softmax function is used to predict a probability value for each word in the vocabulary, which is used to represent the probability that each word contains text semantics;
[0064] Step 14: Select a word set consisting of all words exceeding a certain threshold from the predicted probability value as the topic word set W.
[0065] Specifically, this emb...
specific Embodiment approach 3
[0074] Specific implementation mode three: the difference between this implementation mode and specific implementation mode one or two is that step two specifically includes:
[0075] Step 21. Sentence extraction steps:
[0076] For each word w in the given set of all topic words W, find all sentences containing w in the second training corpus, and put all the sentences found by all words into the set S, for the set S For each sentence of , obtain the vector representation of the sentence; the method for obtaining the sentence vector representation is: obtain the word embedding of each word in the sentence, and then average these word embeddings in each dimension;
[0077] Find the vector representation of the topic word set.
[0078] Make cosine similarity between the vector representation of the topic word set and each sentence vector in the set S respectively.
[0079] Select the sentence with the highest similarity score in each type of sentence as the sentence to be exp...
PUM
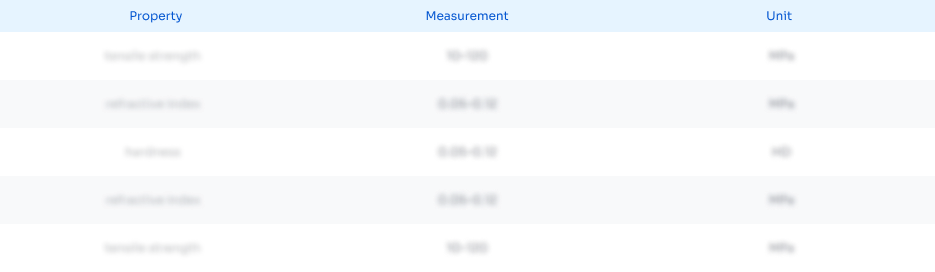
Abstract
Description
Claims
Application Information
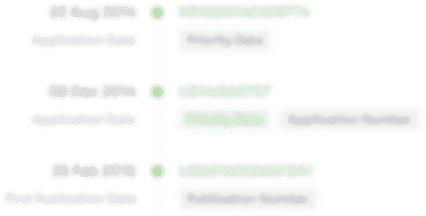
- R&D Engineer
- R&D Manager
- IP Professional
- Industry Leading Data Capabilities
- Powerful AI technology
- Patent DNA Extraction
Browse by: Latest US Patents, China's latest patents, Technical Efficacy Thesaurus, Application Domain, Technology Topic, Popular Technical Reports.
© 2024 PatSnap. All rights reserved.Legal|Privacy policy|Modern Slavery Act Transparency Statement|Sitemap|About US| Contact US: help@patsnap.com