Interpolation grouping sliding gravity center-based nationwide grain consumption prediction method with multiple regression model
A technology of multiple regression model and prediction method, applied in prediction, instrument, data processing application and other directions, can solve the problems of large training error of prediction model, large destructive prediction result, single prediction dependent variable, etc., to achieve smooth prediction error, improve Prediction accuracy, the effect of increasing training data
- Summary
- Abstract
- Description
- Claims
- Application Information
AI Technical Summary
Problems solved by technology
Method used
Image
Examples
Embodiment Construction
[0052] A multivariate regression model national grain consumption prediction method based on interpolation group sliding center of gravity, wherein the grain consumption includes ration consumption, feed grain consumption, seed grain consumption, and industrial grain consumption. Alternatively, the grain consumption is rural grain consumption or urban grain consumption.
[0053] In this embodiment, the grain consumption is taken as an example of rural ration consumption and urban ration consumption:
[0054] The method comprises the steps in turn:
[0055] (1) Obtaining original data: Obtain grain consumption, urban and rural population, urbanization level, urban and rural Engel coefficient, and agricultural product production price index from t=1 to n years as the original sample data.
[0056] The 1981-2015 urban ration consumption, rural ration consumption, urban and rural population, urbanization level, urban and rural Engel coefficient and agricultural product production...
PUM
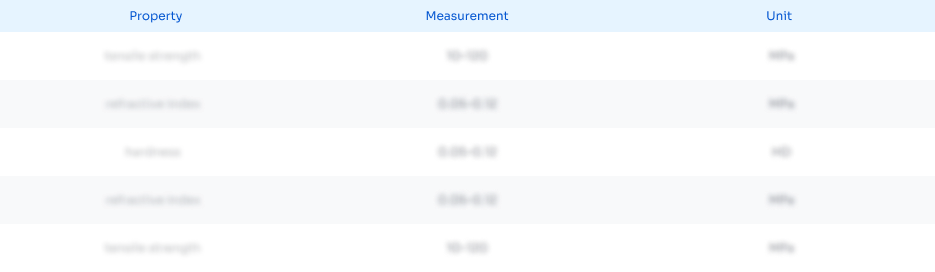
Abstract
Description
Claims
Application Information
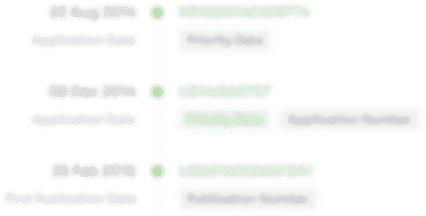
- R&D Engineer
- R&D Manager
- IP Professional
- Industry Leading Data Capabilities
- Powerful AI technology
- Patent DNA Extraction
Browse by: Latest US Patents, China's latest patents, Technical Efficacy Thesaurus, Application Domain, Technology Topic.
© 2024 PatSnap. All rights reserved.Legal|Privacy policy|Modern Slavery Act Transparency Statement|Sitemap