Convex nonnegative matrix factorization method based on subspace clustering
A non-negative matrix decomposition and subspace technology, applied in the fields of data mining, information processing, and data analysis, can solve the problems of subspace clustering and non-negative matrix decomposition collaborative optimization framework, etc., to improve the effect of image clustering, Robustness-enhancing effects
- Summary
- Abstract
- Description
- Claims
- Application Information
AI Technical Summary
Problems solved by technology
Method used
Image
Examples
Embodiment 1
[0063] Embodiment one (with reference to figure 1 )
[0064] Step 1, decompose the original data matrix under the proposed subspace clustering-based convex non-negative matrix factorization framework.
[0065] (1a) Pull each image in the image sample set into a vector to form an m×n original data matrix X, m is the dimension of each sample, and n is the number of samples;
[0066] (1b) Initialize the matrix G of n×l 0 , l×n encoding matrix V 0 It is a non-negative random matrix, l=n s ×n c is the dimension of the learned low-dimensional non-negative subspace, n c is the total number of classes in the database, n s For the number of centers of each cluster, generally set n s =10, the number of iterations t=0.
[0067] (1c) Use the K nearest neighbor algorithm to construct the initial neighbor graph, the number of neighbors K is set to 5, and the initial graph Laplacian matrix L is calculated 0 , where L 0 =D 0 -W 0 , W 0 Represents a symmetric weight matrix, D 0 i...
Embodiment 2
[0095] Embodiment two (with reference to figure 2 )
[0096] Step 1, decompose the original data matrix under the proposed subspace clustering-based convex non-negative matrix factorization framework.
[0097] (1a) Pull each image in the image sample set into a vector to form an m×n original data matrix X, m is the dimension of each sample, and n is the number of samples;
[0098] (1b) Initialize the matrix G of n×l 0 , l×n encoding matrix V 0 It is a non-negative random matrix, l=n s ×n c is the dimension of the learned low-dimensional non-negative subspace, n c is the total number of classes in the database, n s For the number of centers of each cluster, generally set n s =10, the number of iterations t=0.
[0099] (1c) Use the K nearest neighbor algorithm to construct the initial neighbor graph, the number of neighbors K is set to 5, and the initial graph Laplacian matrix L is calculated 0 , where L 0 =D 0 -W 0 , W 0 Represents a symmetric weight matrix, D 0 is...
PUM
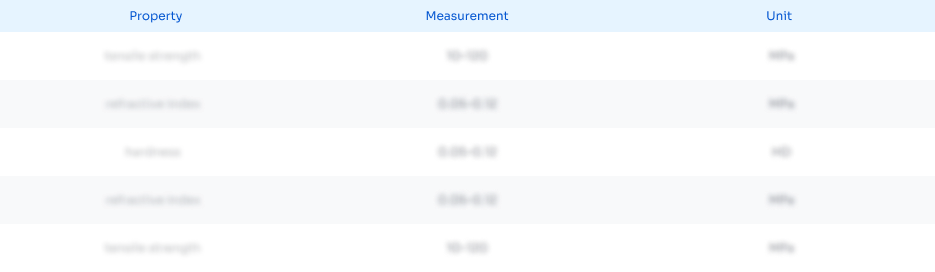
Abstract
Description
Claims
Application Information
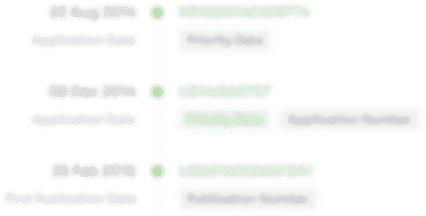
- R&D Engineer
- R&D Manager
- IP Professional
- Industry Leading Data Capabilities
- Powerful AI technology
- Patent DNA Extraction
Browse by: Latest US Patents, China's latest patents, Technical Efficacy Thesaurus, Application Domain, Technology Topic, Popular Technical Reports.
© 2024 PatSnap. All rights reserved.Legal|Privacy policy|Modern Slavery Act Transparency Statement|Sitemap|About US| Contact US: help@patsnap.com