Non-negative matrix factorization method based on low-rank recovery
A technology of non-negative matrix decomposition and low-rank matrix, which is applied in the field of information processing and can solve problems such as non-negative decomposition noise interference
- Summary
- Abstract
- Description
- Claims
- Application Information
AI Technical Summary
Problems solved by technology
Method used
Image
Examples
Embodiment Construction
[0047] see figure 1 , the implementation steps of a preferred embodiment of the present invention are as follows:
[0048] Step 1, perform low-rank sparse decomposition on the original data matrix.
[0049] (1a) Pull each image in the image sample set into a vector to form an m×n original data matrix X, m is the dimension of each sample, and n is the number of samples;
[0050] (1b) Initialize the low-rank matrix as L 0 =X, the sparse matrix is S 0 = 0, the rank of the low-rank matrix is set to r, the sparsity of the sparse matrix is set to k, the relative reconstruction error threshold ε, and the number of iterations t = 0.
[0051] (1c) In order to prevent the singular value of X from gradually degrading, resulting in poor approximation effect of the low-rank approximate matrix of rank r based on the original data matrix X based on bilateral projection, a power correction scheme is adopted, that is, the calculation A bilateral random projection of X instead of a b...
PUM
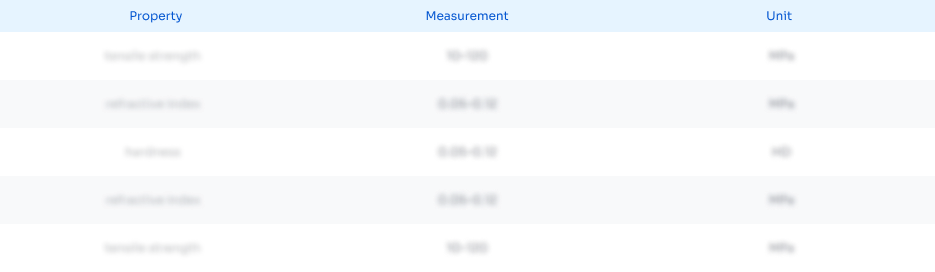
Abstract
Description
Claims
Application Information
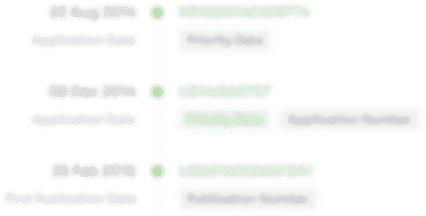
- R&D
- Intellectual Property
- Life Sciences
- Materials
- Tech Scout
- Unparalleled Data Quality
- Higher Quality Content
- 60% Fewer Hallucinations
Browse by: Latest US Patents, China's latest patents, Technical Efficacy Thesaurus, Application Domain, Technology Topic, Popular Technical Reports.
© 2025 PatSnap. All rights reserved.Legal|Privacy policy|Modern Slavery Act Transparency Statement|Sitemap|About US| Contact US: help@patsnap.com