Direct Inverse Reinforcement Learning Using Density Ratio Estimation
A technology of reinforcement learning and density ratio, applied in the field of reverse reinforcement learning system, can solve problems such as complex integral evaluation and inability to solve continuous problems
- Summary
- Abstract
- Description
- Claims
- Application Information
AI Technical Summary
Problems solved by technology
Method used
Image
Examples
preparation example Construction
[0223]
[0224] Consider two sampling methods. One approach is uniform sampling, and the other is trajectory-based sampling. In uniform sampling methods, x is sampled from a uniform distribution defined over the entire state space. In other words, p(x) and π(x) are considered to be uniformly distributed. Then, sample y from the uncontrolled and controlled probabilities to construct D respectively p and D π . In trajectory-based sampling methods, p(y|x) and π(y|x) are used to start from the same state x 0 A trace of the generated state. Then, a pair of state transitions is randomly selected from the trajectory to construct D p and D π . p(x) is expected to be different from π(x).
[0225] For each cost function, the corresponding value function is calculated by solving Equation (4), and the corresponding optimal governed probability is evaluated by Equation (5). In previous methods (Todorov, 2009b, NPL 25), exp(-V(x)) is represented by a linear model, but this is di...
Embodiment approach 2
[0294] Next, Embodiment 2 having characteristics superior to Embodiment 1 in some respects will be described. Figure 12 Differences between Embodiment 1 and Embodiment 2 are schematically shown. As above, and in Figure 12 As shown in (a), Embodiment 1 uses the density ratio estimation algorithm twice and the regularized least squares method. In contrast, in Embodiment 2 of the present invention, the logarithm of the density ratio π(x) / b(x) is estimated using the standard density ratio estimation (DRE) algorithm, and the density ratio π(x) is estimated by using the Bellman equation ,y) / b(x,y) to calculate r(x) and V(x) as reward and value functions, respectively. In more detail, in Embodiment 1, the following three steps are required: (1) estimate π(x) / b(x) through the standard DRE algorithm; (2) estimate π(x,y) / b through the standard DRE algorithm (x,y), and (3) calculate r(x) and V(x) by regularized least squares using the Bellman equation. In contrast, Embodiment 2 use...
PUM
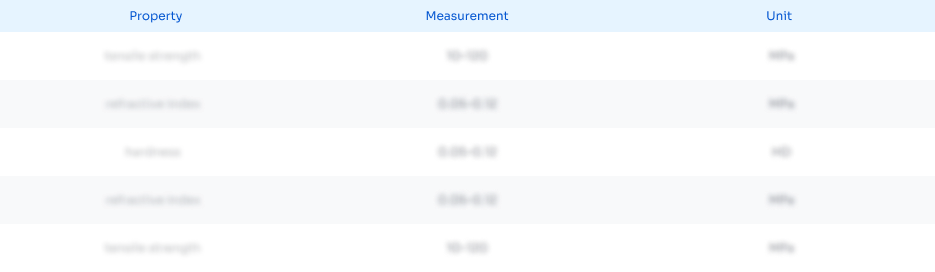
Abstract
Description
Claims
Application Information
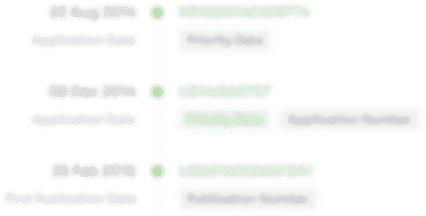
- R&D Engineer
- R&D Manager
- IP Professional
- Industry Leading Data Capabilities
- Powerful AI technology
- Patent DNA Extraction
Browse by: Latest US Patents, China's latest patents, Technical Efficacy Thesaurus, Application Domain, Technology Topic, Popular Technical Reports.
© 2024 PatSnap. All rights reserved.Legal|Privacy policy|Modern Slavery Act Transparency Statement|Sitemap|About US| Contact US: help@patsnap.com