Electroencephalogram signal recognition method based on metric transfer learning
An EEG signal and transfer learning technology, applied in the field of EEG signal recognition based on metric transfer learning, can solve the problems of insufficient generalization ability of the learning model, reduce the classification performance of the transfer learning model, and lose distribution information, etc. Similar to the distance between samples, reducing the distance between samples, and improving the effect of transfer learning
- Summary
- Abstract
- Description
- Claims
- Application Information
AI Technical Summary
Problems solved by technology
Method used
Image
Examples
Embodiment Construction
[0033] The following will clearly and completely describe the technical solutions in the embodiments of the present invention with reference to the accompanying drawings in the embodiments of the present invention. Obviously, the described embodiments are only some, not all, embodiments of the present invention. Based on the embodiments of the present invention, all other embodiments obtained by persons of ordinary skill in the art without making creative efforts belong to the protection scope of the present invention.
[0034] like figure 1 As shown, the implementation of the present invention mainly includes four steps: (1) Map the samples into the shared subspace, and simultaneously perform marginal probability distribution alignment and conditional probability distribution for the labeled EEG source domain data and the unlabeled EEG target domain data. Align distribution; (2) Construct graph Laplacian; (3) Calculate propagation error of metric matrix A by using Mahalanobis...
PUM
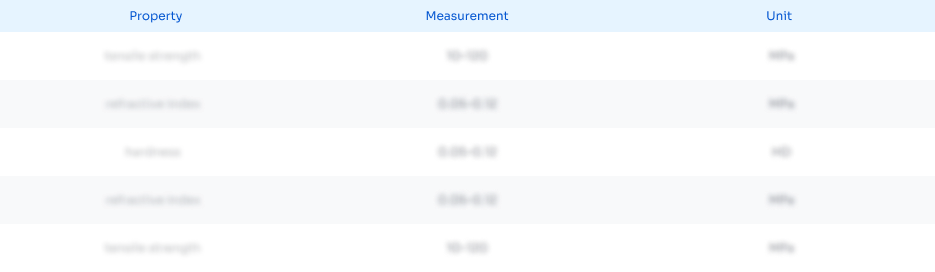
Abstract
Description
Claims
Application Information
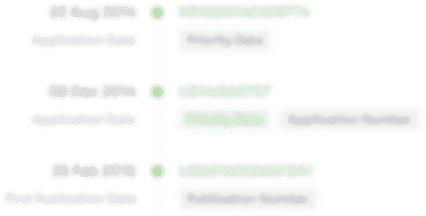
- R&D Engineer
- R&D Manager
- IP Professional
- Industry Leading Data Capabilities
- Powerful AI technology
- Patent DNA Extraction
Browse by: Latest US Patents, China's latest patents, Technical Efficacy Thesaurus, Application Domain, Technology Topic, Popular Technical Reports.
© 2024 PatSnap. All rights reserved.Legal|Privacy policy|Modern Slavery Act Transparency Statement|Sitemap|About US| Contact US: help@patsnap.com