Zero-sample classification method based on low-rank representation and manifold regularization
A technology of low-rank representation and classification method, applied in computer parts, character and pattern recognition, complex mathematical operations, etc., can solve problems such as low classification accuracy, and achieve the effect of enhancing description ability and improving accuracy
- Summary
- Abstract
- Description
- Claims
- Application Information
AI Technical Summary
Problems solved by technology
Method used
Image
Examples
Embodiment Construction
[0025] The Attribute Pascal and Yahoo (aPY) dataset contains 32 categories, of which 20 categories are visible categories for training and 12 categories are unseen categories for testing. Each sample has 64 attribute information. This embodiment uses the aPY data set to illustrate the method proposed by the present invention. In order to make the purpose, features and advantages of the present invention more obvious and understandable, the methods in the embodiments of the present invention will be clearly and completely described below in conjunction with the accompanying drawings in the embodiments of the present invention. Obviously, the following described The embodiments are only some of the embodiments of the present invention, but not all of them. Based on the embodiments of the present invention, all other embodiments obtained by persons of ordinary skill in the art without making creative efforts belong to the protection scope of the present invention.
[0026] see ...
PUM
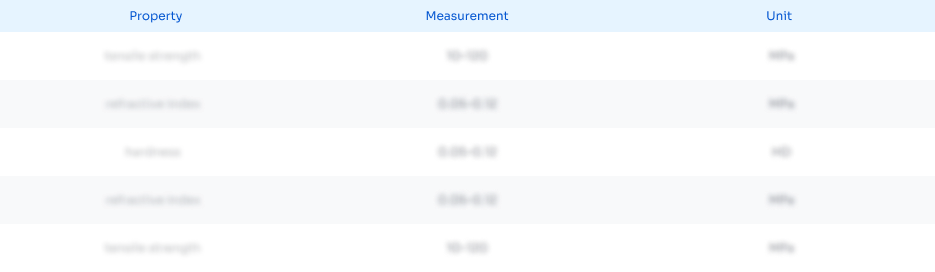
Abstract
Description
Claims
Application Information
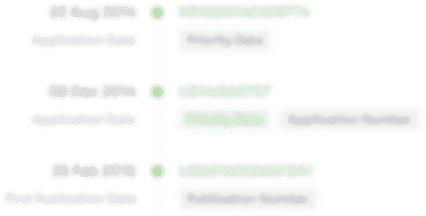
- R&D Engineer
- R&D Manager
- IP Professional
- Industry Leading Data Capabilities
- Powerful AI technology
- Patent DNA Extraction
Browse by: Latest US Patents, China's latest patents, Technical Efficacy Thesaurus, Application Domain, Technology Topic.
© 2024 PatSnap. All rights reserved.Legal|Privacy policy|Modern Slavery Act Transparency Statement|Sitemap