Automatic speech recognition method based on random depth delay neural network model
An automatic speech recognition and neural network model technology, applied in speech recognition, speech analysis, instruments, etc., can solve the problems of limiting neural network learning ability, model parameter growth, gradient disappearance, etc., to solve overfitting and gradient disappearance, Enhanced modeling ability, the effect of strong modeling ability
- Summary
- Abstract
- Description
- Claims
- Application Information
AI Technical Summary
Problems solved by technology
Method used
Image
Examples
Embodiment Construction
[0036] The technical solutions of the present invention will be further described below in conjunction with the drawings and embodiments.
[0037] An automatic speech recognition method based on the stochastic deep time-delay neural network (TDNN-SD) model, which fully considers the respective advantages of stochastic depth and TDNN, and embeds stochastic depth into TDNN. As a long-term dependent modeling model, TDNN has higher computational efficiency and training time than recurrent neural networks. By embedding random depth into TDNN, that is, in the original TDNN, for each TDNN layer with upper and lower frame splicing, a random deep network is introduced to enhance the modeling ability and robustness of the network, and solve the problem of overcrowding in the training process. Fitting and gradient disappearance problems, thereby improving the accuracy of speech recognition.
[0038] A typical speech recognition system consists of feature extraction, acoustic model, lang...
PUM
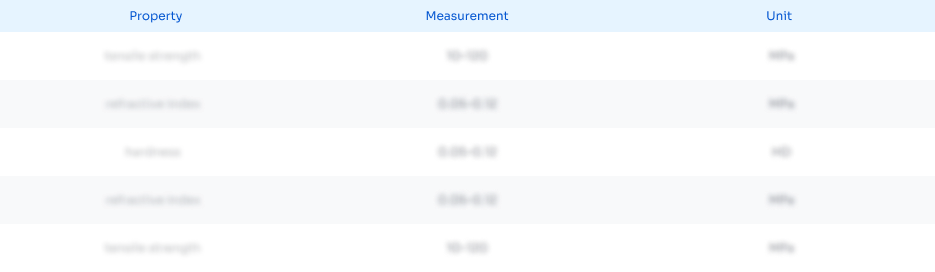
Abstract
Description
Claims
Application Information
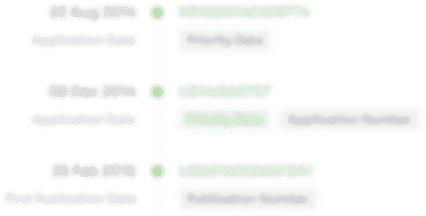
- R&D Engineer
- R&D Manager
- IP Professional
- Industry Leading Data Capabilities
- Powerful AI technology
- Patent DNA Extraction
Browse by: Latest US Patents, China's latest patents, Technical Efficacy Thesaurus, Application Domain, Technology Topic, Popular Technical Reports.
© 2024 PatSnap. All rights reserved.Legal|Privacy policy|Modern Slavery Act Transparency Statement|Sitemap|About US| Contact US: help@patsnap.com