A sign language video translation method based on the fusion of a time domain convolution network and a cyclic neural network
A convolutional network and neural network technology, applied in natural language translation, special data processing applications, instruments, etc., can solve problems such as difficult to learn the correspondence between actions and word semantics, difficult to learn videos, and affect the effect of recognition
- Summary
- Abstract
- Description
- Claims
- Application Information
AI Technical Summary
Problems solved by technology
Method used
Image
Examples
Embodiment Construction
[0051] In this example, if figure 1 As shown, a sign language video translation method based on the fusion of time-domain convolutional network and cyclic neural network is to fully extract the spatial and temporal features in sign language video, effectively learn the features of key actions with high recognition, and effectively Avoid being interfered by factors such as the signer's body shape, sign language speed, and sign language habits during the model learning process. Its steps include:
[0052] First, the original sign language video is preprocessed to extract sign language video features; then two different network structures (time-domain convolutional network TCN and bidirectional recurrent neural network BGRU) are used simultaneously to encode continuous sign language video features and output each slice word Generated probability; then the output of the middle layer of the sub-network is spliced and sent to the fusion network (FL) to learn and generate a word s...
PUM
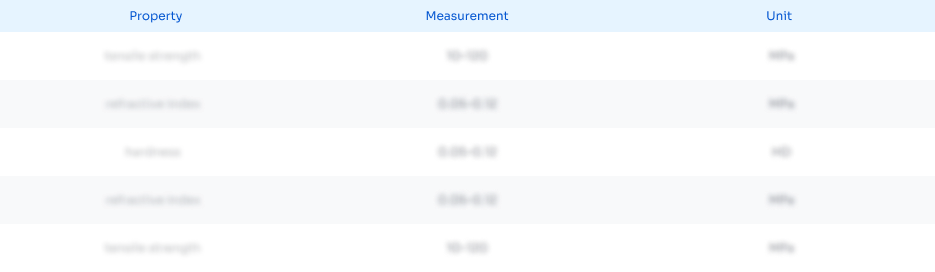
Abstract
Description
Claims
Application Information
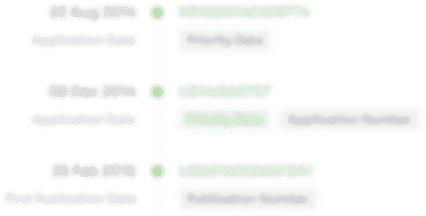
- R&D Engineer
- R&D Manager
- IP Professional
- Industry Leading Data Capabilities
- Powerful AI technology
- Patent DNA Extraction
Browse by: Latest US Patents, China's latest patents, Technical Efficacy Thesaurus, Application Domain, Technology Topic, Popular Technical Reports.
© 2024 PatSnap. All rights reserved.Legal|Privacy policy|Modern Slavery Act Transparency Statement|Sitemap|About US| Contact US: help@patsnap.com