A fast segmentation method for image semantics based on multi-level network
A technology of semantic segmentation and level network, applied in the field of image semantic segmentation and deep learning, can solve the problem that the speed and accuracy of image semantic segmentation cannot be balanced, and achieve the effect of reducing redundancy, improving efficiency and reducing the amount of parameters.
- Summary
- Abstract
- Description
- Claims
- Application Information
AI Technical Summary
Problems solved by technology
Method used
Image
Examples
Embodiment Construction
[0032] In order to make the object, technical solution and advantages of the present invention clearer, the present invention will be described in further detail below in combination with specific implementation and accompanying drawings.
[0033] In order to solve the problem of slow image semantic segmentation in the prior art, the present invention provides a fast image semantic segmentation method based on a multi-level network.
[0034] Below, the most preferred embodiments of the present invention will be described in detail.
[0035] In this embodiment, the image segmentation data set uses the Cityscapes urban street scene data set, which contains 20 category labels (including 1 background label), covering 50 cities in Europe, a total of 5000 finely labeled data sets, of which 2975 are used as The training data set, 500 as the verification data set, and 1525 as the test data set.
[0036] Such as figure 1 As shown, this embodiment includes the following steps:
[003...
PUM
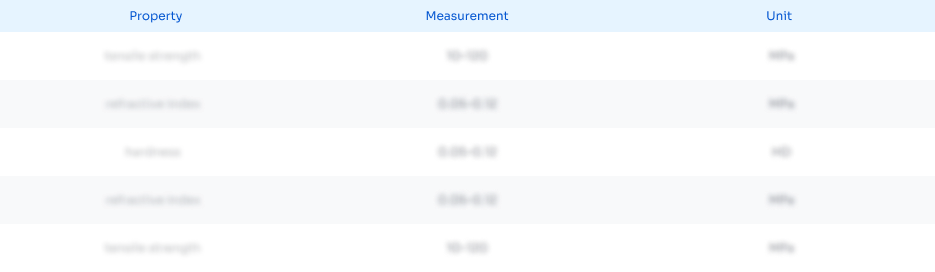
Abstract
Description
Claims
Application Information
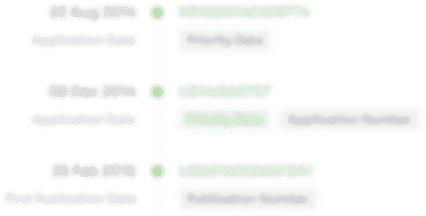
- R&D Engineer
- R&D Manager
- IP Professional
- Industry Leading Data Capabilities
- Powerful AI technology
- Patent DNA Extraction
Browse by: Latest US Patents, China's latest patents, Technical Efficacy Thesaurus, Application Domain, Technology Topic, Popular Technical Reports.
© 2024 PatSnap. All rights reserved.Legal|Privacy policy|Modern Slavery Act Transparency Statement|Sitemap|About US| Contact US: help@patsnap.com