A Semantic Segmentation Method of SAR Image Based on Contour Structure Learning Model
A contour structure and learning model technology, applied in the field of image processing, can solve problems affecting segmentation results, etc., to achieve the effect of improving regional consistency, improving accuracy, and overcoming the blurring of structural features
- Summary
- Abstract
- Description
- Claims
- Application Information
AI Technical Summary
Problems solved by technology
Method used
Image
Examples
Embodiment Construction
[0060] The present invention will be further described below in conjunction with the accompanying drawings and embodiments.
[0061] refer to figure 1 , the SAR image semantic segmentation method based on the outline structure learning model of the present invention comprises the following steps:
[0062] Step 1, using the sketch model of the SAR image to extract the sketch map.
[0063] Input the synthetic aperture radar SAR image, and use the sketch model of the synthetic aperture radar SAR image to obtain the sketch of the SAR image. The specific process is as follows:
[0064] Step 1.1, within the range of [100, 150], randomly select a number as the total number of templates;
[0065] Step 1.2, construct a template of edges and lines composed of pixels with different directions and scales, use the direction and scale information of the template to construct an anisotropic Gaussian function, and calculate the weight of each pixel in the template through the Gaussian funct...
PUM
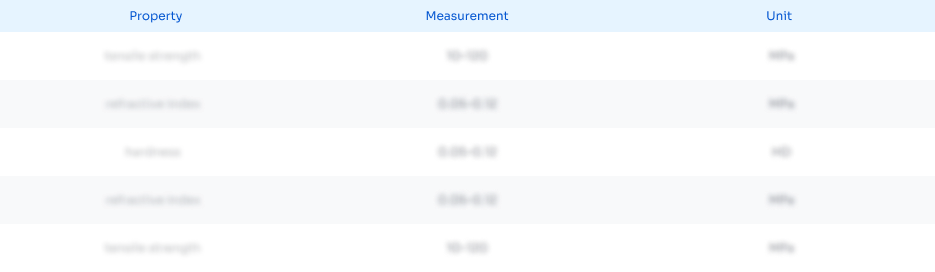
Abstract
Description
Claims
Application Information
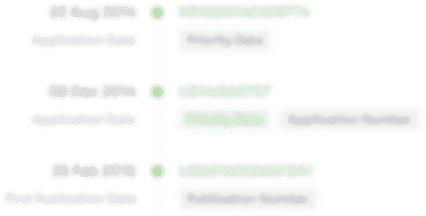
- R&D
- Intellectual Property
- Life Sciences
- Materials
- Tech Scout
- Unparalleled Data Quality
- Higher Quality Content
- 60% Fewer Hallucinations
Browse by: Latest US Patents, China's latest patents, Technical Efficacy Thesaurus, Application Domain, Technology Topic, Popular Technical Reports.
© 2025 PatSnap. All rights reserved.Legal|Privacy policy|Modern Slavery Act Transparency Statement|Sitemap|About US| Contact US: help@patsnap.com